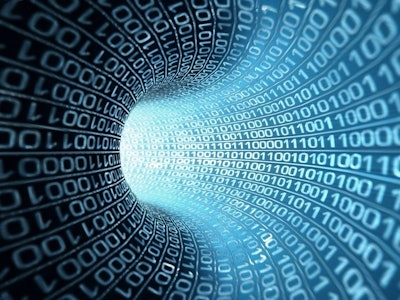
More and more these days we are hearing about big data challenges and opportunities. So what exactly is big data and why should supply chain professionals care about the idea?
There is no single reference standard or definition of big data. However, it is generally taken to mean a collection of data sets that are so large as to be very difficult, if not practically impossible, to manipulate (capture, store, analyze) with traditional tools. Many experts reference the “3-Vs” of big data: large volume, greater velocity and increased variety, first introduced by analyst Doug Laney of Meta Group in 2001. We would add the following characteristics:
- Fully disaggregated: at the raw level, no summaries by product line, market, channel, and so on
- Surprisingly common: often been around for years in extant business systems
Are there examples in the supply chain world? Absolutely. Point-of-sale transactions and RFID data streams come immediately to mind, although for many companies even these do not meet the oft-cited big data threshold of terabytes (trillions) or even petabytes (1000 terabytes) of raw data. More typically one encounters (1) transaction histories (invoices, bills of lading, and so on), (2) inventory records at the stocking location/item code level, and (3) freight rate tables. Such databases can easily reach hundreds of megabytes and include tens of millions of discrete values. Strictly speaking perhaps not big data, but certainly large enough to overwhelm spreadsheets and many database analysis tools. In short, cumbersome to handle but rich enough to yield important insights… if you know how to do it.
And that is the crux of the matter. Once you get beyond the dazzle of sheer totals, how do you apply the old adage of dataèinformationèknowledge? First of all, you need analytical tools that are up to the task. Here are some examples that use allthree of the examples listed above… simultaneously.
Strategic Supply Chain Network Design Tools
Transaction history files serve as a rich source of data for:
- Detailed statistics, graphics, reports and maps useful for analyzing historical demand patterns
- Inputs to rater engines
- Lane-by-lane historical flows for model baseline
- Demand tables for optimization models
- Daily customer demands for dynamic simulation models
Freight rate tables serve as a source of data for:
- Rater engines for optimization and simulation models
- Pattern and outlier analysis
Inventory tables serve as a source of data for:
- Specialized inventory analysis tools
- Baseline comparisons
Transportation Analysis Tools
Transaction history files serve as a source of data for:
- Lane-by-lane historical shipments
- Inputs to rater engines
- Daily customer demands for shipment planning and consolidation algorithms
Freight rate tables serve as a source of data for rater engines
Note these critical observations about the above process:
- The data are not an end in themselves.
- You will not be able to obtain all of the above data from a single source, including Enterprise Resource Planning (ERP) systems. Beware those who assert that ERP compatibility is the magic key to all (or even most) data availability for advanced supply chain analytics-based tools. Treat it as you would other marketing hype… with a large discount factor.
- Corporate databases of the size we are discussing here are notoriously “dirty,” regardless of contrary assertions by your IT folks and vendors. It is essential to have filters that identify and isolate rogue entries… and to know where to look for problems in the first place.
- It is not enough to simply amass basic descriptive statistics (counts, sums, means, variance, frequency distributions), no matter how cleverly they are displayed with visualization tools. Rather, genuine advanced analytics are required to tease relationships and generate managerial insights and prescriptive advice from the data. Always look under the cover when you see the often misused term “advanced analytics.” Descriptive statistics, graphics and maps are unquestionably useful, but do not merit the label “advanced.” Examples of genuine advanced analytics include:
- Prescriptive modeling using mathematical optimization
- Descriptive modeling using stochastic simulation
- Predictive modeling using statistical methods that identify relationships: regression analysis, factor analysis, etc.
Dr. Jeff Karrenbaueri is president of INSIGHT, Inc., a leading supply chain planning solutions provider for the world's foremost companies.
To read more manufacturing and technology news, sign up for our newsletter. You can also follow Manufacturing Business Technology on Twitter @MBTwebsite.