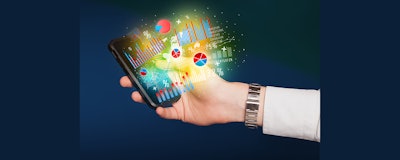
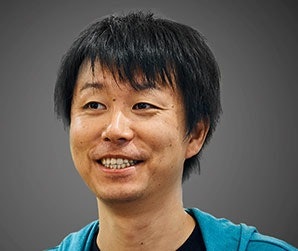
Manufacturers are well-versed in gathering data from their operations, but don’t always know how to use it. Data science automation can provide direction. Dr. Ryohei Fujimaki, CEO and founder, of dotData, joins us to predict how machine learning will change data science over the next year.
1. The pressure to achieve greater return on investment from Artificial Intelligence (AI) and machine learning (ML) initiatives will push more business leaders to seek innovative solutions. While substantial investments are being made in data science across many industries, the scarcity of data science skills and resources limits the advancement of AI and ML projects within organizations. In addition, one data science team is only able to execute several projects a year given the iterative nature of the process and the manual work that goes into data preparation and feature engineering. In 2019, data science automation platforms will cover much wider areas than machine learning automation, including data preparation, feature engineering, machine learning and the production of data science pipelines. These platforms will accelerate data science, executing more business initiatives whilst maintaining the current investments and resources.
2. Transparency and interpretability will become even more important than accuracy. Traditional data science approaches are "black boxes" that result in less actionable business outcomes. Given the current regulatory climate, as it relates to profiling (GDPR, etc.), businesses are demanding increased transparency along with actionability. Current data science processes focus primarily on accuracy and not transparency. In 2019, we will see an emergence of new tools that will enable data scientists to have greater transparency, while sacrificing little in the way of accuracy. This shift to a more “white box” approach to data science will deliver more transparent and accurate models.
3. The democratization of data science and the increasing importance of “citizen” data scientists. Big Data is on the rise, and with it has come a growing demand for skilled data scientists. The shortage of data scientists has created a big challenge for businesses implementing AI and ML initiatives, creating bottlenecks and slowing time-to-production. In 2019, we will begin to see the rise of new data science platforms that will significantly simplify tasks that formerly could only be completed by data scientists. Data science process automation unlock data science for non-data scientists, like Business Intelligence engineers, data engineers, and business analysts, and allows data scientists to focus on complex and high-value projects. Democratization of data science through automation empowers enterprises to not only accelerate but establish the culture of data-driven decision making across the entire organization.
4. Data science tasks will become a 5-minute operation and deliver business values in days. Gone are the days of waiting several months for a data science project. In 2019, we are going to see a transformation in how businesses implement and optimize their AI and machine learning initiatives. New data science automation platforms offer a single, seamless platform that enables companies to accelerate, democratize, and operationalize the entire data science process – from raw data through feature engineering to machine learning – eliminating the most time-consuming and labor- and skill-intensive tasks of data science. As a result, what once took months to complete now will take only days, significantly accelerating time to value for AI and machine learning initiatives.
5. Operationalizing data science will become the next challenge. While business are realizing that AI and machine learning can help drive business transformation, AI and machine learning models can only generate business value when they are fully operational and put into production. The traditional approach to data science is time-consuming and resource-intensive, resulting in many data science projects either chopped or ending up as one-time reports. In the next year, model operationalization as well as model development will be significantly simplified by automation. New data science automation platforms will enable enterprises to deploy, operate and maintain data science processes in production with minimal effort, helping companies maximize their AI and ML investments.