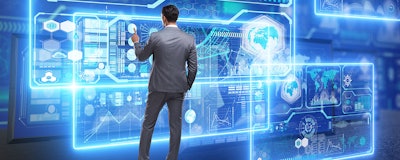
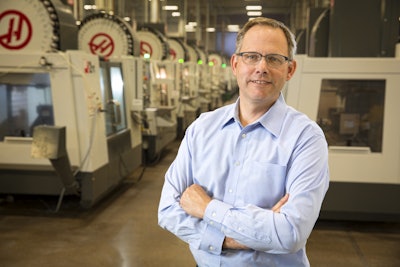
Traditional, large-volume manufacturers often have one goal in mind—reduce costs associated with making high volumes of a single item by producing as many parts as possible in the shortest amount of time. That business model works for high-volume products, particularly if automation can be deployed, but it ignores innovation and the competitive edge created by digital manufacturing. The convergence of software and flexible manufacturing makes quick-turn prototyping and low-volume, on-demand production of custom parts fast and economical. In addition, digital manufacturers capture massive amounts of data across their business from the first customer engagement, through the sales and quoting process, and throughout the manufacturing and fulfillment processes. To take full advantage of all the information, understand the complexity of a high product mix and optimize business, companies need sophisticated analytics that cross business domains.
Data science, a vast discipline encompassing expertise in sophisticated math, engineering, and computer science techniques, typically involves using advanced analytics to mine insights out of data—data that informs business decisions, accelerates growth and improves customer experiences. Much of this data originates from the digital thread that starts with a customer’s first interaction with a digital manufacturer’s website and connects front-end software to automated fulfillment. In Protolabs’ space, front-end software automates design, manufacturability analysis and quoting, then connects it to intelligent, computer-aided manufacturing (CAM) and computer-integrated manufacturing (CIM) hardware on the factory floor. This makes digital manufacturing one of the drivers of Industry 4.0, the union of physical and digital systems through complex software algorithms, artificial intelligence, machine learning and the network of smart industrial devices that comprise the industrial internet of things (IIoT).
Can You Survive Without Data Science?
With the importance of data science, one could then assert the need for a data scientist, a relatively new but growing title at manufacturing companies across the country. Bringing a data scientist on board may seem foreign to manufacturers still operating the old way—targeting reduction of variability and stability of process control to reduce defects and increase productivity. But digital manufacturing companies are leveraging the insights of data crunchers to improve productivity, increase efficiency and reduce waste in an environment of rapidly changing product mix. In a fully flexible environment, machines continue operating optimally, with data-driven survival analysis determining when to pull a machine, a mold, or a tool for maintenance even before it breaks or fails. It is not enough to recognize a process shift while a single product is being produced; it is necessary to recognize shifts that are significant across changing production. When producing small quantities on demand, there is no time or capacity to have undisciplined processes, so predictive analytics becomes a matter of survival.
Introducing Retail-Style Customer Data into the Manufacturing World
While data scientists are relatively new and still less common to manufacturing companies, their work is helping to drive the growth of e-commerce giants like Amazon. Manufacturers that apply retail-style data analytics to their e-commerce function stand to improve their business-to-business online sales and find new revenue opportunities. Evaluating cart abandonment, for example, can help manufacturers identify why customers leave orders behind. Identifying the point in the process when customers get frustrated can narrow down possible root causes, such as uncertainty in logistics, terms or complexity in the checkout process. Such insights into customer paths to completing an order can help improve website designs. Manufacturers that embrace data science and analytics will gain access to insights about customers and processes they simply haven’t had access to before. The result? Much like online retail, improving customers’ B2B e-commerce experiences will boost satisfaction throughout the online ordering process.
Website analytics also can also save manufacturers resources by distinguishing between customers. Some customers are ready to buy but need some help, perhaps in the form of an online chat with industry experts on part manufacturability. Others are casual customers who need minimal attention. Web analytics save customers time as well, streamlining transactional business to avoid contacting—and potentially annoying—customers who know exactly what they want and how to order it. Tailoring the e-commerce experience to serve different demographics helps manufacturers serve customers with what they need, when they need it, allowing manufacturers to not only retain their existing customer base but also attract new customers.
Data—and Capacity—to Grow On
Inversely, manufacturing—which intersects product marketing, segmentation, pricing, production and customer behavior—is also a new field to many data scientists. Before data can be used to optimize revenue or margins for a company or benefits for customers, it’s necessary to first understand the company’s manufacturing technologies. With Protolabs, data scientists need to know the differences between 3D printing and CNC machining, for example, or sheet metal fabrication and injection molding. Next, one needs to understand the customer base, the quantity, the breadth and the segments they serve, such as automotive, medical device, aerospace, robotics or consumer products. This forms the dataset to track new customers, retain existing customers and monitor business with both loyal and infrequent customers.
Analyzing historical data on customer ordering patterns can provide insight for sales forecasts that will help the manufacturer plan for peak periods and avoid capacity problems. By aggregating activity data from all stages of business along with historical trends, capacity can anticipate demand. This enables the manufacturer to continue delivering quickly and producing parts in whatever quantity customers need.
The Future of Data Science in Manufacturing
So, how will digital manufacturers use data science in the future? Machine learning will be critical as companies race to find even better ways to serve customers. Analytics and machine learning will help digital manufacturers identify what capabilities customers will value including price, lead times, quality offerings and other options. Manufacturers that use analytics will enjoy a critical edge that will lead to growing their business.
Rich Baker. Ph.D., is the chief technology officer at Protolabs.