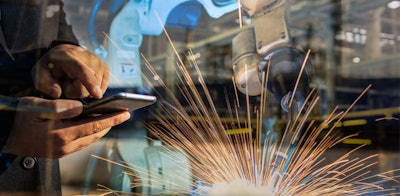
A
N
AL
YT
IC
S
RE
AL
LY
D
O
M
AT
TE
R
Dr
iv
in
g
Di
gi
ta
l T
ra
ns
fo
rm
at
io
n
an
d
th
e
Sm
ar
t M
an
uf
ac
tu
rin
g
En
te
rp
ris
e ANALYTICS
REALLY DO MATTER
Driving Digital Transformation and the
Smart Manufacturing Enterprise
lnsresearch.com
CONNECT:
ANALYTICS REALLY DO MATTER
Driving Digital Transformation and the Smart Manufacturing Enterprise
lnsresearch.com
CONTENTS
SECTION 1:
Critical Upgrade for Industry 4.0: From “Metrics That Matter”to “Analytics That Matter”. . . . . . . . . . . . . . . . . . . 3
SECTION 2:
Closer Look at Operational Architecture Elements . . . . . . . . . . . . . . . . . . . . . . . . . . . . . . . . . . . . . . . . . . . . . . . . . . . . . . . 14
SECTION 3:
Analytics With a Purpose: People . . . . . . . . . . . . . . . . . . . . . . . . . . . . . . . . . . . . . . . . . . . . . . . . . . . . . . . . . . . . . . . . . . . . . . . . . . 20
SECTION 4:
Recommendations for Analytics and Digital Transformation . . . . . . . . . . . . . . . . . . . . . . . . . . . . . . . . . . . . . . . . . . . . . . 25
ACRONYM
QUICK REFERENCE
VIEW ON BLOG
Critical Upgrade for Industry 4.0:
From “Metrics That Matter”
to “Analytics That Matter”
SECTION 1
4
SECTION
TABLE OF
CONTENTS
PAGE
A
N
AL
YT
IC
S
RE
AL
LY
D
O
M
AT
TE
R
Dr
iv
in
g
Di
gi
ta
l T
ra
ns
fo
rm
at
io
n
an
d
th
e
Sm
ar
t M
an
uf
ac
tu
rin
g
En
te
rp
ris
e
1 2
3 4
For the last fourteen years, LNS Research and its predecessors pub-
lished bi-annual research in partnership with MESA Manufacturing
on the use of data in manufacturing. Until now, the research was ti-
tled, “Metrics That Matter.” This year marks an important shift in fo-
cus, examining “analytics that matter.” This evolutionary step in the
study marks an important milestone to align with how companies
conduct their Digital Transformation journey. As companies begin
that journey, we talk a lot about Industrial Internet of Things (IIoT)
platforms and applications that run on them. However, the heart of a
digital enterprise is data and using it to improve enterprise-wide per-
formance. One of the major tools we have to achieve this is analytics.
This ebook introduces:
• The results of the 2018 Analytics that Matter survey and the
unexpected (and interesting) responses; and
• A data and analytics architecture that fits within the Oper-
ational Architecture prescribed by LNS Research and that
helps manufacturers achieve Digital Transformation goals.
Over the last year or two, analytics applications have appeared
from every imaginable source, from large control, IT and cloud
vendors to “one man and my algorithm” start-ups. In the industri-
al world, much of this has been focused on specific applications
such as asset performance management (APM) and other mainte-
nance-related processes. For most of operations, the starting point
of data use today is simple metrics with nice displays to show the “up
to the moment” state of affairs. In reality, very little “live data” moves
from operational systems into outside plant data stores. However,
that’s about to change and it’s what will drive deep value — prescrip-
tive control from live analytics. This is the opportunity that drives the
shift in focus from metrics to analytics.
A Better Focus for Today’s Industrial Enterprise
OPERATIONAL ARCHITECTURE by LNS Research is
a critical component of Industrial Transformation. It extends
traditional enterprise architecture, to holistically manage
the convergence of information technology and operations
technology. It’s an approach to align people, process, and
technology in context of the value chain, and in support of
enterprise Strategic Objectives.
Click to learn more about Operational Architecture
5
SECTION
TABLE OF
CONTENTS
PAGE
A
N
AL
YT
IC
S
RE
AL
LY
D
O
M
AT
TE
R
Dr
iv
in
g
Di
gi
ta
l T
ra
ns
fo
rm
at
io
n
an
d
th
e
Sm
ar
t M
an
uf
ac
tu
rin
g
En
te
rp
ris
e
1 2
3 4
Metrics Will Remain
On time delivery Successful NPI WIP inventory turnover
Net profit margin OEE First pass yield
Capacity utilization Average changeover time None of the above
Average manufacturing cycle time Downtime vs. operating time Number products produced per plant
∆ in output/throughput Mean cost per unit excluding materials
Workplace injuries Number SKUs produced Average supplier incoming quality
60% 37% 27%
37% 26%60%
37% 30% 6%
37% 29%
43% 36% 24%
39% 32% 23%
6
SECTION
TABLE OF
CONTENTS
PAGE
A
N
AL
YT
IC
S
RE
AL
LY
D
O
M
AT
TE
R
Dr
iv
in
g
Di
gi
ta
l T
ra
ns
fo
rm
at
io
n
an
d
th
e
Sm
ar
t M
an
uf
ac
tu
rin
g
En
te
rp
ris
e
1 2
3 4
The focus of the survey was across manufacturing execu-
tives responsible for operational technology (OT), but the
survey was open to all. To the extent possible, we eliminat-
ed vendor responses which resulted in a good cross-sec-
tion of reliable respondents. At the time of publication
(June 2018) the total number of responses for this analysis
was approximately 350. If you are a manufacturer and hav-
en't yet taken the analytics that matter survey, please par-
ticipate now. Doing so will give you access to continued
research coverage of this and related topics.
Some of the essential starting points for Digital Trans-
formation are somewhat dependent on the specific indus-
try. The analytics that matter survey captured responses
across many industries, and this report examines the data
according to three main segments:
discrete manufacturing, pro-
cess manufacturing, and
batch (or hybrid).
Demographics
GEOGRAPHY
COLOR BY HQ LOCATION
REVENUE
COLOR BY COMPANY REVENUE
North America
Europe
Asia / Pacific
Rest of World
Small: <$250M
Medium: $250M-$1B
>$1B
37%
29%
34%
50%
26%
11%
13%
JOB TITLE
Senior Executive
Director
Manager
Consultant
All Others
12%
23%
27%
22%
16%
INDUSTRY
Discrete Manufacturing
Process Manufacturing
Batch Manufacturing
44%
28%
28%
DISCIPLINE / ROLE
Operations
Engineering
Information Technology
Quality
Maintenance
Research and
Development
All Others
26%
20%
20%
6%
5%
4% 19%
7
SECTION
TABLE OF
CONTENTS
PAGE
A
N
AL
YT
IC
S
RE
AL
LY
D
O
M
AT
TE
R
Dr
iv
in
g
Di
gi
ta
l T
ra
ns
fo
rm
at
io
n
an
d
th
e
Sm
ar
t M
an
uf
ac
tu
rin
g
En
te
rp
ris
e
1 2
3 4
0100010111011000100101
0100010111011000100101
0100010111011000100101
0100010111011000100101
0100010111011000100101
0100010111011000100101
0100010111011000100101
0100010111011000100101
10110111010010010001
10110111010010010001
01001000110110111010010010001
10110111010010010001
10110111010010010001
010010001010010001
010010001
010010001
0100010111011000100101
0100010111011000100101
0100010111011000100101
0100010111011000100101
0100010111011000100101
0100010111011000100101
0100010111011000100101
0100010111011000100101
10110111010010010001
10110111010010010001
01001000110110111010010010001
10110111010010010001
10110111010010010001
010010001010010001
010010001
010010001
Before the days of integrated manufacturing operations manage-
ment (MOM) systems, automation and supervisory control were
made up of many “point solutions,” single function programs with
a tendency for limited communication and from multiple vendors.
Although MOM has taken over much of the functionality, a lot of
these solutions persist in many plants. To get at least some value out
of analytics, the first step is to collect relevant data from centralized
systems plus the older systems dotted around the plant. As always,
there is a broad range of responses, from companies with advanced
automation, integration and analytics, to those that still use Excel or
paper forms for most of the reporting.
Today’s Norm: Beyond Point Solutions
Plant scheduling
Workflow / process management
Plant analytics
Statistical process control (SPC)
Quality management
Operations / manufacturing intelligence
Production execution
Data historian
Plant / process simulation
HMI / visualization
Mobile apps for visualization
IIoT platform
Predictive modeling
Recipe management
Advances process control (APC)
Process analytical technology (PAT)
MOM / MES
0% 10% 20% 30% 40% 50% 60% 70%
59%
58%
65%
63%
57%
55%
55%
49%
49%
48%
48%
47%
47%
43%
42%
42%
41%
Manufacturing Applications Adopted
8
SECTION
TABLE OF
CONTENTS
PAGE
A
N
AL
YT
IC
S
RE
AL
LY
D
O
M
AT
TE
R
Dr
iv
in
g
Di
gi
ta
l T
ra
ns
fo
rm
at
io
n
an
d
th
e
Sm
ar
t M
an
uf
ac
tu
rin
g
En
te
rp
ris
e
1 2
3 4
One of the clear trends that we see is that companies are looking at
their Strategic Objectives and realize that some form of Digital Trans-
formation is a necessity to stay competitive for the next 3-5 years.
Companies that are not already highly digitalized recognize that the
first step to a digital future is data gathering. There are many ways
to initial data gathering, from SCADA, to building an IIoT platform
across the enterprise.
When we drill down to examine just process manufacturing, we
learn that systems implemented is considerably different than the
manufacturing industry overall. Cuts like this can help manufac-
turers better to focus on what will bring maximum benefit to them
when making Digital Transformation plans. In the following pages,
we will look at a broad cross-section of results and what they mean
to manufacturers.
Today’s Norm: Beyond Point Solutions (Cont.)
How does the IIoT impact your business today?
We are still investigating the impact
Don't understand/know about IIoT
We understand/are aware but see
no impact at this time
We understand/are aware and we
see value to our operations
We understand/are aware and our
customer demands are driving us
We understand/are aware and have
already seen dramatic impact
0% 5% 10% 15% 20% 25% 30% 35% 40%
27%
18%
18%
14%
13%
9%
9
SECTION
TABLE OF
CONTENTS
PAGE
A
N
AL
YT
IC
S
RE
AL
LY
D
O
M
AT
TE
R
Dr
iv
in
g
Di
gi
ta
l T
ra
ns
fo
rm
at
io
n
an
d
th
e
Sm
ar
t M
an
uf
ac
tu
rin
g
En
te
rp
ris
e
1 2
3 4
Let’s start with “who is in charge?” We’re encouraged to see that
corporate and plant analytics programs are for the most part led by
senior staff. This structure aligns well with the LNS Research Digital
Transformation framework, which strongly encourages C-level ex-
ecutives to own the process and to lead by setting and monitoring
Strategic Objectives. Ownership of the analytics program is a great
start. However, for success with the analytics program, it needs to
be integrated throughout the entire business from sensor to board-
room. More importantly, it must involve all the right people, from
those who can benefit to those needed to implement.
Analytics Today: Who, What, Where?
22%
19%
18%
CEO / COO
Plant management
Chief digital officer
14%
CIO
10%
9%
6%
2% 1%
Analytics
department head
Non C-level
department head
Other C-level
executive Other C-level
executive
Automation / engineering leaders
Other Other
Corporate Analytics: Responsible Role
16%
15%
CEO / COO
Manufacturing
IT leaders
15%15%
12%
Plant
management
Analytics
department head
Chief
digital officer
8%
7%
4%
CIO
Plant Analytics: Responsible Role
10
SECTION
TABLE OF
CONTENTS
PAGE
A
N
AL
YT
IC
S
RE
AL
LY
D
O
M
AT
TE
R
Dr
iv
in
g
Di
gi
ta
l T
ra
ns
fo
rm
at
io
n
an
d
th
e
Sm
ar
t M
an
uf
ac
tu
rin
g
En
te
rp
ris
e
1 2
3 4
Next, we should consider how the organization uses analytics.
Today we see predominantly traditional processes aimed at quality,
forecasting, productivity and Operational Excellence. Those that fall
under the umbrella of Digital Transformation are as yet less popular
– social media, traceability, cross plant comparisons, and the like.
That’s not surprising since analytics for digital change is absolutely
part of the IIoT journey, and it’s an essential part of any IIoT platform.
Today, many manufacturers are experimenting with IIoT platforms
using only analytics as the initial use cases. Asset performance
management (APM) has been a long-time low-hanging fruit for
IIoT implementation, and in reality, it only uses data collection and
analytics to implement current generation APM.
Analytics Today: Who, What, Where? (Cont.)
Big Data Analytics, Collaboration, and Mash-Up Apps
Connectivity and Data Model
ANALYTICS & APPSANALYTICS & APPSANALYTICS & APPS
SUPPLIERS OPERATIONS CUSTOMERS & PRODUCTS
EDGE ANALYTICS
AND APPLICATIONS
EDGE ANALYTICS
AND APPLICATIONS
EDGE ANALYTICS
AND APPLICATIONS
EDGE ANALYTICS
AND APPLICATIONS
EDGE ANALYTICS
AND APPLICATIONS
EDGE ANALYTICS
AND APPLICATIONS
EDGE ANALYTICS
AND APPLICATIONS
EDGE ANALYTICS
AND APPLICATIONS
EDGE ANALYTICS
AND APPLICATIONS
CONNECTIVITY-CENTRIC OPERATIONAL ARCHITECTURE
46% 43% 39% 36% 34%
Improving
manufacturing quality
Better forecasts of a
production plant
Operational Excellence
programs
Continuing manufacturing
process improvement
Improved customer
service and support
TOP 5 USES OF ANALYTICS IN THE MANUFACTURING ENTERPRISE
11
SECTION
TABLE OF
CONTENTS
PAGE
A
N
AL
YT
IC
S
RE
AL
LY
D
O
M
AT
TE
R
Dr
iv
in
g
Di
gi
ta
l T
ra
ns
fo
rm
at
io
n
an
d
th
e
Sm
ar
t M
an
uf
ac
tu
rin
g
En
te
rp
ris
e
1 2
3 4
Much has been done inside the plant to collect data (historians,
MOM, SCADA, etc.) and, as we have seen, companies have gener-
ated lots of metrics to run the manufacturing business better. Some
but not all of those who need access to timely information to do their
job can access it with existing systems. While that’s very encouraging
at the plant level, it’s less so when we consider the wider world of
information sharing. Analytics and the IIoT promise much more, not
just in the plant but across the enterprise and beyond.
As we move into the IIoT world, analytics will be a part of the tool-
kit available to those running a digital business. However, it will not
be everything. When we look at analytics offerings from a multitude
of vendors today, the one common trait is that they rarely use ana-
lytics to genuinely control plants and the business. Metrics are great
for diagnostic and descriptive analytics, but the enterprise can only
realize rich value when it moves to predictive and prescriptive forms.
IIoT is a Principal Starting Point
Current state of Industrial Analytics Program
Budgeted within 1 year
Fully implemented
In pilot implementation
Planned within 3 years
Not implemented
0% 5% 10% 15% 20% 25% 30%
29%
25%
25%
19%
9%
30%
27%
25%
22%
21%
19%
4%
Supervisors
Operations managers
Plant level operators
IT / Business analysts
Plant managers
Quality personnel
Plant / control engineers
Maintenance personnel
Machine level operators
Process / improvement staff
Supply chain planners
Line / area level operations
Business unit managers
C-level executives
Don't know
54%
41%
39%
38%
35%
34%
34%
31%
Which roles have access to analyzed info from
plant within timeframe required to make
appropriate decisions?
12
SECTION
TABLE OF
CONTENTS
PAGE
A
N
AL
YT
IC
S
RE
AL
LY
D
O
M
AT
TE
R
Dr
iv
in
g
Di
gi
ta
l T
ra
ns
fo
rm
at
io
n
an
d
th
e
Sm
ar
t M
an
uf
ac
tu
rin
g
En
te
rp
ris
e
1 2
3 4
The use of descriptive and diagnostic analytics is much as we
would expect; about one-third of manufacturers use these an-
alytics (in reality, old-fashioned business intelligence (BI) and
enterprise manufacturing intelligence (EMI) across the enter-
prises. The surprise comes with predictive analytics, with a
level of deployment very close to the first two. That degree
of adoption means that companies are trying hard to increase
their analytics skills and, in some ways, it correlates with anoth-
er interesting finding: nearly 40% of companies claim that they
have sufficient data scientists to manage analytics needs.
IIoT is a Principal Starting Point (Cont.)
ANALYTICS FRAMEWORK
DESCRIPTIVE DIAGNOSTIC PREDICTIVE PRESCRIPTIVE
What
happened
What will
happen
What action
to take
Why it
happened
TRADITIONAL VIEW OF ANALYTICS
Descriptive Diagnostic Predictive Prescriptive None
Production
Supply Chain
Finance
Engineering
Corporate quality
Customer relations
55%
50%
45%
40%
35%
30%
25%
20%
15%
10%
5%
0%
Business Analytics Sophistication
Descriptive Diagnostic Predictive Prescriptive None
Production
Inventory / Logistics
Maintenance
Quality
50%
45%
40%
35%
30%
25%
20%
15%
10%
5%
0%
Industrial Analytics Sophistication: Plant Operations and Related
LowVALUE High
PastTIME Future
EasyTECHNICAL
FEASIBILITY
Hard
13
SECTION
TABLE OF
CONTENTS
PAGE
A
N
AL
YT
IC
S
RE
AL
LY
D
O
M
AT
TE
R
Dr
iv
in
g
Di
gi
ta
l T
ra
ns
fo
rm
at
io
n
an
d
th
e
Sm
ar
t M
an
uf
ac
tu
rin
g
En
te
rp
ris
e
1 2
3 4
0100010111011000100101
0100010111011000100101
0100010111011000100101
0100010111011000100101
0100010111011000100101
0100010111011000100101
0100010111011000100101
0100010111011000100101
10110111010010010001
10110111010010010001
01001000110110111010010010001
10110111010010010001
10110111010010010001
010010001010010001
010010001
010010001
CSS37%
HTML
SQL
We suspect that these two data points come from the use of BI and
EMI applications in relatively sophisticated ways and have little cor-
relation with Big Data analytics that are a fundamental part of Digital
Transformation and the IIoT.
The extensive dialogue about the value of data scientists and an-
alytics that takes place in the heady heights of the data intelligence
industry does not seem to have reached the calms of manufacturing
enterprise IT departments. Manufacturers should closely examine
the skills they will need throughout the Digital Transformation pro-
cess and then honestly and objectively evaluate existing capabilities.
IIoT is a Principal Starting Point (Cont.)
Manufacturers should closely examine the skills
they will need throughout the Digital Transformation
process and then HONESTLY AND OBJECTIVELY
EVALUATE EXISTING CAPABILITIES.
We have a STRONG TEAM OF DATA SCIENTISTS
that will not require much help
Closer Look at Operational
Architecture Elements
SECTION 2
15
SECTION
TABLE OF
CONTENTS
PAGE
A
N
AL
YT
IC
S
RE
AL
LY
D
O
M
AT
TE
R
Dr
iv
in
g
Di
gi
ta
l T
ra
ns
fo
rm
at
io
n
an
d
th
e
Sm
ar
t M
an
uf
ac
tu
rin
g
En
te
rp
ris
e
1 2
3 4
One of the trends over the last few years has been the advent of
cloud computing, first to the enterprise and now gradually to the
plant. While the LNS IIoT platform shows Cloud as the main data
block, in reality the world has evolved to “Cloud and Edge.” When
we examine where a company runs various types of analytics, we can
determine its highest level architecture.
Cloud and Edge: What, Where, Why
Finance is the most conservative department with little Cloud
and predominantly corporate data center use. Most manufacturers
we have spoken with find it fascinating that production leads the way
into the Cloud. Where plant analytics is concerned little changes, but
it’s encouraging that 25% of companies have some analytics running
on public Cloud.
Corporate Analytics: Location
Public cloud
Private cloud
Corporate data center
Plant data center
Edge device / asset
None
PRODUCTION ENGINEERING CORPORATE QUALITY CUSTOMER RELATIONSSUPPLY CHAIN FINANCE
23% 32% 22% 20% 24%21%
40% 42% 42% 45% 41%56%
14% 13% 18% 15% 13%11%
5% 6% 5% 12% 8%5%
6% 6% 9% 7% 12%6%
23% 12% 9% 12% 13%8%
Industrial Analytics: Location
Public cloud
Private cloud
Corporate data center
Plant data center
Edge device / asset
None
PRODUCTION MAINTENANCE QUALITYINVENTORY / LOGISTICS
22% 30% 25% 22%
38% 44% 48% 37%
28% 31% 32% 38%
8% 10% 8% 7%
4% 3% 4% 5%
24% 15% 10% 13%
16
SECTION
TABLE OF
CONTENTS
PAGE
A
N
AL
YT
IC
S
RE
AL
LY
D
O
M
AT
TE
R
Dr
iv
in
g
Di
gi
ta
l T
ra
ns
fo
rm
at
io
n
an
d
th
e
Sm
ar
t M
an
uf
ac
tu
rin
g
En
te
rp
ris
e
1 2
3 4
It’s hard to decide where to run what in an unchanging operational
state but going through Digital Transformation makes life much more
complex. As a company builds out a new Operational Architecture
there are enterprise architecture changes required for Digital
Transformation. Furthermore, the organization must make a series
of data location choices. This is especially true to support analytics,
but some infrastructure choice must happen early on. The company
should consider a basic data architecture to make these choices.
For many organizations, a new way of thinking about IIoT data
management has undoubtedly helped. We now see the discussion
moving away from “everything in the Cloud” to the much more
flexible “from Cloud to Edge.” Of course, it’s important to remember
that between Cloud and Edge there are other places where data can
reside, but we can’t discuss them until we first find the Edge!
Cloud and Edge: What, Where, Why (Cont.)
Defining the Edge is difficult because it depends heavily on the
company’s initial viewpoint. A company that makes computers for
corporate data centers will view computers and systems that sit in the
plant as Edge systems. They would include systems like manufacturing
operations management (MOM), data historians, SCADA, HMI, PLCs
and so on. On the other hand, a pump manufacturer would perceive
the pump's controllers as Edge systems and everything higher up the
control hierarchy and beyond is “on-premise” or “I don’t care.”
Either of these perspectives is an excellent starting point to
manage data placement if the architecture is to remain somewhat
faithful to the ISA-95 standard that has served operational technology
leaders and technologists well over the last few decades. In other
words, everything that sits below the MOM layer (Level 3 in ISA-95)
consider Edge, and MOM systems as on-premise, Cloud or wherever
they happen to reside.
IT EXECUTIVE
VIEW OF THE WORLD
Cloud
On-Premise
Edge
OPERATIONS EXECUTIVE
VIEW OF THE WORLD
Cloud
On-Premise
Edge
ROLE AFFECTS PERCEPTION OF EDGE
SMART CONNECTED ENTERPRISE
17
SECTION
TABLE OF
CONTENTS
PAGE
A
N
AL
YT
IC
S
RE
AL
LY
D
O
M
AT
TE
R
Dr
iv
in
g
Di
gi
ta
l T
ra
ns
fo
rm
at
io
n
an
d
th
e
Sm
ar
t M
an
uf
ac
tu
rin
g
En
te
rp
ris
e
1 2
3 4
While Edge to Cloud is important for the highest level Operation-
al Architecture, there is much more to be done than just deciding
where data will sit. All data must be useful and accessible to applica-
tions that need it. Rather than a deep-dive on application and data
architecture, let’s explore just a few data requirements for analytics.
This is simply a cursory view and any Digital Transformation program
requires a detailed study of data architecture.
First consider how the company wants to use the data; for analyt-
ics several dimensions are important:
• Data location: Edge (device/asset), on-premise (plant data
center, enterprise data center), Cloud
• Data speed: decision time and bandwidth constraints,
streaming and protocols, historical (pointers or replicated)
• Data type: structured, semi-structured, unstructured
• Analytics model: statistical, first principles, artificial intelli-
gence (AI), machine learning (ML)
• Purpose: display, long-term improvement, direct feedback to
control system, improve people processes
Data and Analytics Architecture in a Digital World
Fast
Latent
Precise
Ambiguous
Structured
Semi-structured
Unstructured
SPEED AND
QUALITY OF DATA
TYPES OF
ANALYTICSTYPES OF DATA
Descriptive
Diagnostic
Predictive
Prescriptive
Visualization
DIMENSIONS OF
DATA AND ANALYTICS
18
SECTION
TABLE OF
CONTENTS
PAGE
A
N
AL
YT
IC
S
RE
AL
LY
D
O
M
AT
TE
R
Dr
iv
in
g
Di
gi
ta
l T
ra
ns
fo
rm
at
io
n
an
d
th
e
Sm
ar
t M
an
uf
ac
tu
rin
g
En
te
rp
ris
e
1 2
3 4
When we examine uses none specifically say “analytics,” but many
such as energy efficiency and asset reliability are the low hanging
fruit in the industrial analytics industry. Most of the top IIoT use cases
today aren’t to provide instantaneous feedback to the plant or pro-
cess. Indeed, when we discuss industrial use with analytics vendors,
we rarely see feedback loops. Why? There is a strong fear of analyt-
ics applications setting automatic control. That’s why we see a low
uptake of prescriptive analytics. However, an industrial organization
will only achieve real value when new analytics methods
provide new insights on processes, then feed that information back
into the process itself. Companies can use many applications with
human intervention (such as planned maintenance improvements),
but others can directly affect equipment, design information, prod-
uct flow or production speed; the possibilities are endless.
Data and Analytics Architecture in a Digital World (Cont.)
Remote monitoring
Energy efficiency
Asset reliability
Business model transformation, e.g.
selling capacity instead of products
Production visibility
Quality improvement
Asset and material tracking
Internet enabled products
Traceability and serialization
Customer access to information
Improving safety
Supplier visibility
Improving environmental
performance
0% 5% 10% 15% 20% 25% 30%
Top IIoT Use Cases
28%
27%
25%
24%
17%
8%
22%
15%
6%
6%
4%
13%
22%
Improving manufacturing quality
Better forecasts of a production plant
Operational Excellence programs
Continuing manufacturing
process improvement
Improved customer service and support
Better forecasts of sales
Customer Relationship Management
Understand customer requirements
for new products
Better forecasts of production
across multiple plants
Finding key plant performance parameters
Continuing Asset Performance
(APM) improvement
Alert management across multiple plants
Trading products outside the enterprise
Social media interaction with consumers
We do not share manufacturing
data outside the enterprise
Delivering software upgrades directly
to products in the field
Don't know
Other examples
0% 10% 20% 30% 40% 50%
39%
46%
43%
36%
34%
33%
31%
27%
27%
24%
19%
17%
16%
15%
13%
12%
6%
1%
Analytics to Improve Business and Manufacturing Processes
19
SECTION
TABLE OF
CONTENTS
PAGE
A
N
AL
YT
IC
S
RE
AL
LY
D
O
M
AT
TE
R
Dr
iv
in
g
Di
gi
ta
l T
ra
ns
fo
rm
at
io
n
an
d
th
e
Sm
ar
t M
an
uf
ac
tu
rin
g
En
te
rp
ris
e
1 2
3 4
As we move into more advanced fields such as Edge analytics and
Big Data analytics in the Cloud, data abstraction and cleansing will
become ever more important. Managing local analytics at the deep
Edge (e.g., on a motor controller) and directly feeding the control
system changes the dynamics of data. We often talk about the “four
V's” of data – velocity, volume, variety, and veracity. In the deep Edge
example, we want to be able to store fast and voluminous data locally
for a short time while we conduct local analytics. Longer term deci-
sion making will take place at a higher level in the data stack (perhaps
in MOM or in the Cloud) and require reduced velocity and volume
through consolidation. Similarly, the longer-term feedback loops will
not require much speed or volume, but they must deliver the neces-
sary feedback to the system as designed.
Data and Analytics Architecture in a Digital World (Cont.)
Aggregate Data
STORAGE
EDGE CLOUD
Prescriptive Advice
ANALYTICS APP
MACHINE
PLC
Live Data In
Control Command
EXTENDED PERSPECTIVE OF CONTROL
Analytics With a Purpose: People
SECTION 3
21
SECTION
TABLE OF
CONTENTS
PAGE
A
N
AL
YT
IC
S
RE
AL
LY
D
O
M
AT
TE
R
Dr
iv
in
g
Di
gi
ta
l T
ra
ns
fo
rm
at
io
n
an
d
th
e
Sm
ar
t M
an
uf
ac
tu
rin
g
En
te
rp
ris
e
1 2
3 4 Six Sigma
IT'S EITHER
“IN AND DONE”...
Percentage of
companies that are
fully implemented:
... OR “WE DON'T
CARE ABOUT IT.”
Percentage of companies
with no plans:
53% 49% 47% 36%
34% 40% 40% 47% 48%
25%
Operational
Excellence
ISO 9000/9001 Lean TQM
Demand
Driven
Manufacturing
Lean Startup ISO 14001 OSHAS 18001 Agile / Scrum
When talking analytics, data management and Digital Transforma-
tion, it’s easy to forget about the role of people. To understand this,
we examined the use of continuous improvement methods and how
organizations apply digital tools to or with them.
Analytics and People - Continuous Improvement
When talking analytics, data management and
Digital Transformation, IT’S EASY TO FORGET
ABOUT THE ROLE OF PEOPLE.
22
SECTION
TABLE OF
CONTENTS
PAGE
A
N
AL
YT
IC
S
RE
AL
LY
D
O
M
AT
TE
R
Dr
iv
in
g
Di
gi
ta
l T
ra
ns
fo
rm
at
io
n
an
d
th
e
Sm
ar
t M
an
uf
ac
tu
rin
g
En
te
rp
ris
e
1 2
3 4
Lean and Six Sigma are very popular traditional methods for continu-
ous improvement. Toyota started the trend, and many have followed
the rigorous path these people-centric processes prescribe. Many
have achieved truly continuous improvement to the point where in-
cremental improvements are barely detectable, but that is no reason
for letting go — the domino effect of these programs is dramatic
across companies.
Analytics and People - Continuous Improvement (Cont.)
WE CARE ABOUT
TRADITIONAL
IMPROVEMENT
METHODS...
Percentage of
companies that are
fully implemented:
Shift Meetings Standardized
Work
5 Whys SPC / Quality
Metrics
5S Production
Leveling
Continuous
Flow
Kaizen Kanban Value
Stream
Mapping
Plan Do
Check Act
Takt Time TPM /
OEE
Gemba DMAIC Poka
Yoke
Andon SMED
45% 41% 38% 36% 34% 31% 30%
29% 27% 27%28% 22% 22% 19% 19% 18% 15% 14%
23
SECTION
TABLE OF
CONTENTS
PAGE
A
N
AL
YT
IC
S
RE
AL
LY
D
O
M
AT
TE
R
Dr
iv
in
g
Di
gi
ta
l T
ra
ns
fo
rm
at
io
n
an
d
th
e
Sm
ar
t M
an
uf
ac
tu
rin
g
En
te
rp
ris
e
1 2
3 4
ANDONPOKA YOKE5S
DMAICGEMBAKAIZEN5 WHYS
There is tremendous resistance to change because of how successful
these programs have been. Blackbelts and sensei’s are highly valued
and do, to an extent, maintain a level of self-aggrandizement that
hinders change. Encouraging and accepting change is the very thing
that has made these programs so successful, yet the leaders resist it.
LNS published research on transforming Lean through digital pro-
cesses, but that approach isn’t always welcome. Many companies
already have successful continuous improvement (CI) programs but
few want to enhance them with digital tools.
Analytics and People - Continuous Improvement (Cont.)
LEAN SIX SIGMA TQM
24
SECTION
TABLE OF
CONTENTS
PAGE
A
N
AL
YT
IC
S
RE
AL
LY
D
O
M
AT
TE
R
Dr
iv
in
g
Di
gi
ta
l T
ra
ns
fo
rm
at
io
n
an
d
th
e
Sm
ar
t M
an
uf
ac
tu
rin
g
En
te
rp
ris
e
1 2
3 4
The research results reveal an interesting correlation between CI
programs and digital tools; the effect is exactly the opposite of
what typical CI leaders believe. In fact, we discovered two close-
ly related correlations about those who digitally transformed their
continuous improvement programs compared to traditionalists.
Those that digitally transformed CI are much more likely to be:
• Using advanced analytics such as predictive and prescriptive
• Running analytics in the Cloud and at the Edge
Analytics and People - Continuous Improvement (Cont.)
...BUT WE
DON'T WANT
TO DO IT
DIGITALLY.
Percentage of
companies with
NO PLANS to
implement
digital tools:
TPM / OEE
DMAIC
Takt TimePlan Do Check Act
Poka Yoke Andon SMEDGemba
61% 61%
Value Stream MappingKanban
Shift Meetings
Kaizan
Standardized Work 5 Whys SPC / Quality Metrics 5S Production Leveling Continuous
Flow
45% 45%43% 50% 51%
53%
46% 46%
58% 52% 48% 52% 55%
59% 56% 58%
Recommendations
for Analytics
and Digital Transformation
SECTION 4
26
SECTION
TABLE OF
CONTENTS
PAGE
A
N
AL
YT
IC
S
RE
AL
LY
D
O
M
AT
TE
R
Dr
iv
in
g
Di
gi
ta
l T
ra
ns
fo
rm
at
io
n
an
d
th
e
Sm
ar
t M
an
uf
ac
tu
rin
g
En
te
rp
ris
e
1 2
3 4
Start at the Beginning: Architecture and Edge
BIG DATA MODEL
COMPUTE + STORAGE
INDUSTRIAL OPERATIONS
INDUSTRIAL ANALYTICS + APPS
PRODUCTION
BUSINESS
ASSET
CONNECTIVITY C
ON
NEC
TIV
ITY
EDG
E
ON-PREMISE
CLOUD
TIME
SERIES
UNS
TRU
CTU
RED
STRUCTURED
DEVELOPMENT ENVIRONMENT
DE
VEL
OPM
ENT
EN
VIR
ON
ME
NT
FIRST
PRINCIPLES
STATISTICS -
BASED
ARTIFICIAL
INTELLIGENCE (AI)
/ MACHINE
LEARNING (ML)
Companies have been very successful with the LNS Research Dig-
ital Transformation framework to-date, despite the fact that until
now it didn’t adequately provide for metrics and analytics. At the
same time, new analytics vendors continue to appear at an alarming
rate. Most are what we used to call “point solutions,” good at col-
lecting data from existing sources and analyzing (hence the name).
We shoehorned them in without much thought for the future.
The new LNS Research take on Operational
Architecture based on the IIoT platform views
analytics in the same context as all other ap-
plications. It also supports the concept of
Cloud to Edge without implying any difference
between them. The definition of Edge leans
towards a hardware-centric view of the enter-
prise – any system that is below a plant data
center (or corporate one if no plant data cen-
ter exists) is considered part of the Edge. That’s
not a hard and fast rule, but excellent guidance
to further the discussion about Operational
Architecture with distributed applications.
GUIDE TO OPERATIONAL ARCHITECTURE
Common Data, Apps, and Analytics
27
SECTION
TABLE OF
CONTENTS
PAGE
A
N
AL
YT
IC
S
RE
AL
LY
D
O
M
AT
TE
R
Dr
iv
in
g
Di
gi
ta
l T
ra
ns
fo
rm
at
io
n
an
d
th
e
Sm
ar
t M
an
uf
ac
tu
rin
g
En
te
rp
ris
e
1 2
3 4
The main point is that we don’t differentiate between analytics run-
ning at the Edge from those running in the Cloud. Operational Archi-
tecture is primarily software-based, and applications and analytics
can run anywhere in the corporate architecture that makes sense.
This approach means you can build the Operational Architecture
without concern for hardware limitations. As an example, a company
could decide to provide sufficient processing power in a PLC to run
local analytics; that might be cost-effective and fit with the analytics
goals, but it doesn’t preclude it from running analytics elsewhere as
long as there is a logical connection to the architecture without be-
ing tied to hardware.
If a company wants to change its world with analytics, it must
embrace all stages of Digital Transformation.
Start at the Beginning: Architecture and Edge (Cont.)
Aggregate Data
STORAGE
EDGE CLOUD
Prescriptive Advice
ANALYTICS APP
PLC
0100010111011000100101
0100010111011000100101
0100010111011000100101
0100010111011000100101
0100010111011000100101
0100010111011000100101
0100010111011000100101
0100010111011000100101
10110111010010010001
10110111010010010001
01001000110110111010010010001
10110111010010010001
10110111010010010001
010010001010010001
010010001
010010001
IT'S TEMPTING FOR “operations people” to skip the first
two stages of the Digital Transformation framework; do-
ing so makes it difficult to set the right path. Aligning with
Strategic Objectives is a fundamental requirement for any
enterprise that wants to succeed. When top management
sets the direction and translates it into near-term and long-
term goals, it sets the stage to drive success with Digital
Transformation. They don’t simply say, “let’s go digital.”
To the contrary, enterprise leadership is setting transfor-
mation goals and demonstrating genuine commitment to
industrial transformation.
Commitment must extend to more than just the C-suite:
anyone and everyone that’s involved in making the company
better in any way must buy-in to the journey. Operational Ex-
cellence, the focus of continuous improvement for technolo-
gy, processes, and people (employees and customers), is a
critical part of the Digital Transformation journey. Certainly,
digitalizing continuous improvement programs can help ac-
celerate improvements from Digital Transformation, and we
offer a special word to and about continuous improvement
(CI) leaders. Those who lead CI are usually deeply committed
to manual processes; these experts must be on-board as
key enablers for digital CI, and hence Digital Transformation.
STRATEGIC OBJECTIVES AND
OPERATIONAL EXCELLENCE
28
SECTION
TABLE OF
CONTENTS
PAGE
A
N
AL
YT
IC
S
RE
AL
LY
D
O
M
AT
TE
R
Dr
iv
in
g
Di
gi
ta
l T
ra
ns
fo
rm
at
io
n
an
d
th
e
Sm
ar
t M
an
uf
ac
tu
rin
g
En
te
rp
ris
e
1 2
3 4
Operational Architecture: Common Data, Apps, and Analytics
The world of Digital Transformation has moved rapidly in the last
two years. Many more LNS Research program members are putting
teams in place to look at what Digital Transformation means to their
business and what they should do about it. Many quickly realize that
they a have a lot of work to do across the enterprise to take advan-
tage of analytics’ potential. Although every company starts from a
different level of capabilities maturity, it is quite clear that a key el-
ement of Digital Transformation is an Operational Architecture that
unites and aligns the key components of a digital enterprise.
For that very reason, LNS Research includes Operational Archi-
tecture in its Digital Transformation framework (discussed earlier in
this ebook). At this juncture, we introduce a guide to Operational
Architecture for manufacturers considering the journey. While the
final Operational Architecture for every manufacturer is quite dis-
tinct, the components and intersections described here are a great
starting point to fuel the internal enterprise dialogue for all. In future
research we will examine each element in greater detail; for now we
offer a brief introduction.
INDUSTRIAL OPERATIONS
Industrial operations is what manufacturing is all about — the actu-
al equipment, processes and people that add value to the business.
Quite simply, operations improvement is the main objective of Digi-
tal Transformation, including:
• Improving asset performance through better
maintenance and planning;
• Increasing production throughput;
• Increasing first pass yield;
• Faster feedback and insight to personnel;
• More and more accurate information to the business; and
• Delivering high-quality data to the digital systems
such as analytics.
INDUSTRIAL OPERATIONS
PRODUCTION
BUSINESS
ASSET
29
SECTION
TABLE OF
CONTENTS
PAGE
A
N
AL
YT
IC
S
RE
AL
LY
D
O
M
AT
TE
R
Dr
iv
in
g
Di
gi
ta
l T
ra
ns
fo
rm
at
io
n
an
d
th
e
Sm
ar
t M
an
uf
ac
tu
rin
g
En
te
rp
ris
e
1 2
3 4
Operational Architecture: Common Data, Apps, and Analytics (Cont.)
COMPUTE AND STORAGE
Many manufacturers have production and business processes that
produce enormous amounts of data. Most of the time, the vast majori-
ty of that data isn’t used for profit. Traditionally, the only data collected
from, and sent to equipment is for control purposes; the digital world
requires much more. Connectivity will be enabled through smart de-
vices with IP (Ethernet) capability, and more performance data will be
stored. Additionally, the whole world outside the plant will become a
source of data, and a place to which data needs to be sent.
To achieve capability maturity with Big Data the Operational Ar-
chitecture must support data everywhere in the enterprise and be-
yond. Some of that data needs to be close to the process, while some
should reside in the Cloud and accessible globally. In designing the
data and connectivity architecture, the enterprise should consider
current and future needs. For general perspective and discussion,
we define three layers of data: Cloud, on-premise, and Edge. Cloud
could be split between public and private, on-premise could be split
multiple ways (for example by site or in enterprise and plant levels),
and Edge should be defined according to the manufacturer’s needs.
Often Edge will be a plant-level data and compute server, but some
companies will want to define Edge as close to control equipment.
Future research on the topic will refine the definition and details of
the storage architecture.
In addition to data, the hardware computing architecture must
match execution requirements. There’s no point in having lots of
storage yet insufficient power to run sophisticated analytics and
other applications. Indeed, access to public Cloud computing pow-
er can be quite beneficial for things like simulation which usually
requires enormous numbers of computer cores to deliver suffi-
ciently fast simulation.
COMPUTE + STORAGE
CONNECTIVITY C
ON
NEC
TIV
ITY
EDG
E
ON-PREMISE
CLOUD
30
SECTION
TABLE OF
CONTENTS
PAGE
A
N
AL
YT
IC
S
RE
AL
LY
D
O
M
AT
TE
R
Dr
iv
in
g
Di
gi
ta
l T
ra
ns
fo
rm
at
io
n
an
d
th
e
Sm
ar
t M
an
uf
ac
tu
rin
g
En
te
rp
ris
e
1 2
3 4
Operational Architecture: Common Data, Apps, and Analytics (Cont.)
INDUSTRIAL ANALYTICS AND APPS
Finally, at the summit of the Operational Architecture is where new
things get done. In this ebook, we have examined how manufacturers
can benefit from analytics, and now we define what they must do to
design a hardware and software architecture to support the promise of
analytics. Many types of analytics run on multiple levels of the Opera-
tional Architecture. We see a variety of technologies like those shown
here, plus it supports the levels of analytic sophistication from diagnos-
tic (simply looking at what is happening), to prescriptive (controlling
the process and business), and most importantly, supporting the peo-
ple who run the business from top to bottom (or operator to CEO).
BIG DATA MODEL
TIME
SERIES
UNS
TRU
CTU
RED
STRUCTURED
BIG DATA MODEL
TIME
SERIES
UNS
TRU
CTU
RED
STRUCTURED
INDUSTRIAL ANALYTICS + APPS
DEVELOPMENT ENVIRONMENT
DE
VEL
OPM
ENT
EN
VIR
ON
ME
NT
FIRST
PRINCIPLES
STATISTICS -
BASED
ARTIFICIAL
INTELLIGENCE (AI)
/ MACHINE
LEARNING (ML)
INDUSTRIAL ANALYTICS + APPS
DEVELOPMENT ENVIRONMENT
DE
VEL
OPM
ENT
EN
VIR
ON
ME
NT
FIRST
PRINCIPLES
STATISTICS -
BASED
ARTIFICIAL
INTELLIGENCE (AI)
/ MACHINE
LEARNING (ML)
BIG DATA MODEL
Once the organization provides for “compute and storage” capabil-
ities, it can move on to delivery of capabilities. The Big Data model
must be able to handle all types of data used in a digital enterprise. We
categorize data three ways:
• Structured, which comes from traditional databases and
equipment;
• Time series, which is very specific to manufacturing and in-
cludes continuously changing and event-based data in spe-
ciality databases (often data historians); and
• Unstructured data, such as video, weather and all sorts of in-
formation that has not typically been used in manufacturing
but will enhance analytics and application development as
Digital Transformation continues into the future.
As companies continue to define their Operational Architecture in
greater detail, it will be interesting to see how they use new types of
data in the different levels of the storage hierarchy. For example, us-
ing augmented reality (AR) / virtual reality (AR) in the plant is already
becoming a reality, but the infrastructure to handle it efficiently still
needs work.
31
SECTION
TABLE OF
CONTENTS
PAGE
A
N
AL
YT
IC
S
RE
AL
LY
D
O
M
AT
TE
R
Dr
iv
in
g
Di
gi
ta
l T
ra
ns
fo
rm
at
io
n
an
d
th
e
Sm
ar
t M
an
uf
ac
tu
rin
g
En
te
rp
ris
e
1 2
3 4
Recommendations
We keep saying it and have no intention of stopping — Digital
Transformation is a “must” for industrial organizations to survive
and succeed today and into the future. Analytics capabilities are
growing quickly, and the number of vendors selling IIoT platforms
and the apps and analytics to go with them is rapidly accelerating.
One clear outcome of our analytics that matter study is that there
are gains to be had just by using analytics. Applying analytics within
a digital framework means the benefits will continue to multiply.
Manufacturers that want to take a deeper dive and compare
their own capabilities should contact LNS Research. Each organiza-
tion’s specific capabilities and objectives lend a unique perspective
to the research. Doing nothing now will in most cases leave the
manufacturer behind the competition. Take the following steps to
do more than just stay in the game:
1. Set ambitious objectives for the company, or for a division of a
large company; it is not necessary to jump in with both feet,
but you do have to get your feet wet.
2. Choose a Digital Transformation team, headed by a top opera-
tional executive and sponsored by the C-suite or board.
3. Ensure that CI and Operational Excellence is well represented;
this isn’t an exercise to replace Operational Excellence with
Digital Transformation. Aligning these major transformational
processes is how companies will achieve the fastest gains.
4. Try an IIoT platform — don’t commit, but do try. Focus on
applications and analytics that drive true improvements in
plant processes. This is an opportunity to test the connectiv-
ity and ensure that plant-level data is available.
5. Plan for the long-term; for example, set a goal to achieve specific
targets by the start of the next fiscal year, or by the next annual
general meeting, and for a digital enterprise that delivers long-
term strategic value within the next three to five years.
lnsresearch.comConnect:
ACRONYM
QUICK REFERENCE
VIEW ON BLOG
Presented by:
ANALYTICS REALLY DO MATTER
Driving Digital Transformation and the Smart Manufacturing Enterprise
Author:
Andrew Hughes
Principal Analyst
[email protected]
© LNS Research, 2018. All Rights Reserved.
License to distribute this research
report has been granted to: