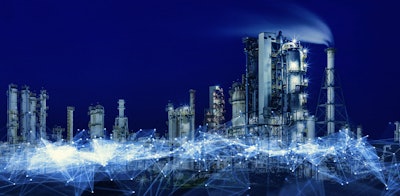
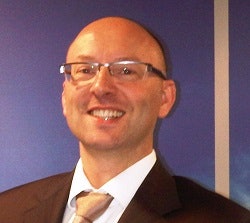
In the world of IIoT, data is considered to be the new oil. But its full potential will only be unleashed if operational context can be taken into account when analyzing, monitoring or predicting operational performance. Shedding necessary light onto time-series data through dynamic contextualization enables you to take your processes into the highest gear of operational excellence, and your organization into the future.
Factories today are capturing and storing an enormous amount of data directly or indirectly related to the production process. All this captured data typically ends up in business applications serving specific operational purposes. Some of the data is stored in historical archives, other data goes into the quality information system, maintenance management system, incident management system, etc. Often all this data is unconnected, and so the question becomes: Can you find the relation between the data in various repositories?
Is all the data captured in your organization illuminating your production facilities so you can operate faster? In many cases we see that if a factory is run by experts, even with all their knowledge in their minds, they're basically running the factory in the dark. Self-service analytics of time-series data already sheds light on operational performance. But if you have all the available contextual information available, captured during production and leveraged from other applications, you have a much better visibility to your operations. You can drive much faster over an illuminated highway than in the dark. That’s also the case when contextual information helps you to analyze faster, run more efficiently, and have more yield.
Making use of your sensor-generated time-series data creates a lot of possibilities to improve operational performance through use of self-service analytics, as this practical example shows.
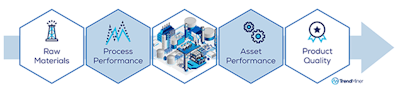
Practical Use Cases: Avoid Distillation Column Trips
In a continuous production process, the distillation columns in a specialty chemicals plant are used for separating methyl acetate and methanol by adding water on top to break the azeotrope. A temperature controller near the bottom of the column is designed to make sure no methyl acetate is being entrained. A pressure spike recently occurred, which negatively impacted production and quality. The goal is to find out whether this was a single incident or if it happened before, and if so, whether a root cause of the issue could be found.
To check if the situation had occurred before, the pressure profile was used for finding similar behavior in all historical time-series data. By performing a similarity search and overlaying the results, a very similar event (>90 percent match) was found, which happened a few months ago. By overlaying the results, the pattern of the events shows the same shape, which naturally led the engineers to believe they might be due to similar cause.
Instead of searching manually for potential root causes, the recommendation engine was used to get suggestions from the self-service analytics solution. The subject matter expert can easily iterate to find the insights he or she is looking for. In this case, quite rapidly, a number of interesting tags were suggested by the software for the engineer to assess further. It became apparent that the combination of high reflux with insufficient boil-up or steam to the reboiler (steam evaporation) during the start-up phase of the column were the main causes of the spike.
Through the recommendation engine, it also became clear that a higher tray temperature in the column is a nice early indicator for the pressure spike. A monitor is set up that will alert the production engineer as soon as the temperature starts dropping, so timely actions can be taken and the consequences mitigated.
The analysis has shown that an undesired combination of process conditions will lead to unstable column operation, which in turn leads to bad separation and bad bottom product quality. The monitors that have been set up,will give the engineers and operators sufficient time to react and avoid these situations going forward. Each event would realistically lead to several hours of lost production and degraded quality. At an average throughput of 25t/h, this leads to more than 100 tons of off-spec product saved per event, and inversely, 100 tons of additional on-spec produced by avoiding the event altogether.
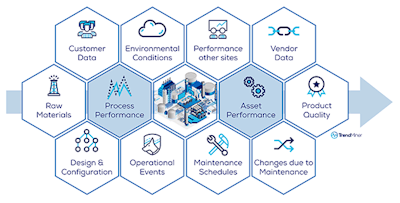
Smarter Analytics With Contextual Data
The use of captured time-series data, in combination with the knowledge of your process and asset experts, makes you operate faster and improves overall performance. As said before, direct and indirect operational data is being captured by various business applications. If this data can be linked to the time-series data during trend analysis even more operational improvements can be gained.
A first logical addition of contextual information is tying the quality test data from the laboratory to the process data. Especially in case of batch production, where the context of a batch (such as batch number, cycle time, etc.) can be linked to the test data from the laboratory. In this way, each specific batch run is not only tied to its process data but also its own quality data. This extra linked information enables quicker assessment of the best runs for creating golden batch fingerprints to monitor future batches. It also helps to collect the underperforming batches for starting your analysis to improve the production process.
Leveraging All Contextual Information
Whether you have a continuous production process or work in batches, a wide range of contextual data can shed new light on your operational performance. Think of captured events during the production process, such as maintenance stops, process anomalies, asset health information, external events, production losses, etc. Also degrading performance of equipment can indicate that the product quality will be impacted, which can be used to assure product quality. All this contextual information helps to better understand operational performance and give new starting points for optimization projects when using your advanced analytics platform.
Concluding Thoughts
Many companies in the process manufacturing market already leverage their time-series data for improving operational performance. The best results are gained when subject matter experts can analyze the data themselves with use of self-service advanced analytics software. When data from other business applications can be tied to the time-series data, new insights can be gained. Data in those business applications give new starting points for process improvements. The insights may lead to monitors capturing new events for future deeper assessment of the production process, and thus resulting in a continuous improvement cycle. This approach will help reducing costs related to waste, energy, and maintenance and increase yield with quality products.
About the Author:
Edwin van Dijk is VP Marketing, TrendMiner.