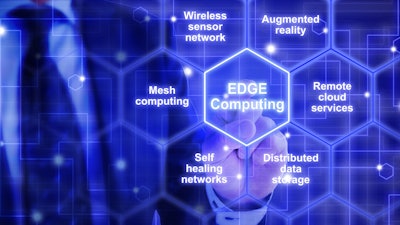
As manufacturers seek to streamline their businesses, they want to gain more insight into the health of their equipment, with the goals of keeping it running smoothly, ensuring optimal performance, and reducing unexpected downtime.
Automation has long been used ito help achieve these goals, and in recent years, advances in key technologies—including artificial intelligence (AI) and Internet of Things (IoT)—have allowed manufacturers to take advantage of more sophisticated industrial use cases for automation, such as bin picking, and collaborative and autonomous mobile robots.
Today, new types of assets are taking automation even further, as machines such as robotic welding arms and injection molding machines use hundreds of sensors that combine with existing data sources to improve overall equipment effectiveness (OEE). In the case of a robotic arm, for example, the machine data spans a large number of sensor and actuator measurements from the robotic arm itself, and external sources that indicate other operational and environmental conditions (e.g., line speed, job style, ambient temperature and humidity).
The process of joining these technologies with AI-based IoT technologies is playing an increasingly important role in delivering tangible business value throughout the manufacturing environment.
One downside of so much automation is a heavier dependence on machinery/robots to perform action. For some lines, if one robot goes down, the entire line goes down, making it critical to detect the health of these assets and possible signs of failure prior to an issue. This is accomplished by understanding what normal behavior of an asset is. By understanding the healthy cycle for a machine, anomalies and signs of failure can more easily be detected within the equipment behavior.
Manufacturers are now seeing AI’s potential to move beyond observing and reacting to machine behavior to looking at overall performance and productivity, including creating a deeper understanding of signs of failure on equipment, cycle times, operator performance, maintenance scheduling and material planning through a 360-degree view of operations. This is achieved by pulling data from programmable logic controllers (PLCs), manufacturing execution systems, and sensors to create a deeper understanding of signs of failure. AI-based IoT technologies is not only allowing for deeper insight into the current and historical behavior and performance of operations, but now enables forward-looking projections on how production lines will run in the future.
Overcoming Challenges
One technology advancement that is helping manufacturers gain this insight is AI device training at the edge. Training a device to process critical data at the network edge via AI can make IoT implementations much more efficient, with greater predictive accuracy and efficacy over solutions trained in the cloud.
In many scenarios, standard IoT analytics collect data from the edge devices and pass them to the cloud for analysis, then back to the device for action. There are two key problems with this approach: cost and latency.
For most IoT and machine learning applications, such as those in manufacturing, latency is not an option because of the carefully orchestrated synchronization between sensors and machines. Cost is also an issue; transmitting all data to the cloud for processing can be too expensive for most manufacturing applications.
The cellular network used by most companies for their IoT implementations can lead to some unexpected costs as usage grows. The cost of using the cloud to store the data also increases as data usage grows. This is where edge device training becomes increasingly important.
Training each device separately at the edge allows the device to learn in the same environment and under the same conditions in which it will operate. By being able to train devices in the actual environment versus training them all similarly in the cloud, and passing down directives from there, performance is naturally improved.
Edge Training in Action
An example from automotive manufacturing best shows the value of training at the edge. The industry leverages a large quantity of robotic welders and robotic material handlers. Across one facility, there may be 1,000 or more robotic arms doing the same job in tandem with fixtures holding parts. Transmitting that data to a cloud environment to train can be costly.
By using AI at the edge, potentially catastrophic maintenance and repair issues can be discovered and mitigated more quickly. Data points can be gathered from assets at rates exceeding once every 100 ms. With AI processing data at the edge, the business could understand the health of its equipment, as well as if there were anomalies or signs of failure. Stakeholders were then alerted when anomalies were detected.
Using AI to train at the edge is a critical part of IoT implementations, especially as they scale. Edge training can help reduce costs and latency, and increase performance for IoT implementations by ensuring equipment is always in peak condition. The cloud will always be an integral part of the IoT, however, and training at the edge and in the cloud should go hand in hand to gain the largest benefit to an edge AI deployment.
Yasser Khan is CEO of One Tech, Inc., a global organization focused on redefining artificial intelligence at the network edge. He is a veteran business executive and serial entrepreneur in the digital transformation space with a focus on business process automation, IIoT and artificial intelligence/machine learning. In 2016, Khan was nominated for “Entrepreneur of the Year” by Ernst & Young LLP.