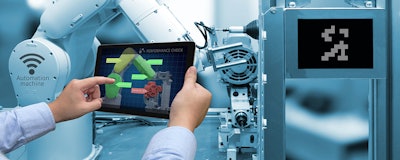
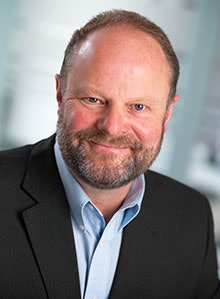
Most manufacturing operations are based on highly automated processes, and their owners and operators are familiar with the regular maintenance and normal failure modes of their equipment. In many plants, if a serious failure occurs which is outside the operator's normal experience, the plant manager inevitably relies on one or two experts who have worked with the process for many years and was probably involved in the original setup or installation of the equipment. We all recognize these individuals; they’re the ‘go to’ people if something goes wrong, and without them, the process can often be down for lengthy periods.
In an increasing number of cases, these specialists are close to retirement age. This poses the question of how best to capture the knowledge accumulated by them over the years so that the next time an unexpected breakdown or process issue occurs (particularly outside office hours), the process isn’t down for an extended period. There is a risk that the specialists are unavailable at the right time, meaning minor issues could quickly become major ones, with obvious effects on the continued operability of a process. This business risk is compounded when a manufacturer has a large number of global manufacturing sites.
Careful succession and contingency planning is one approach to mitigating the effects of loss of expertise and know-how, but increasingly, the model of employing a large number of technical staff to manage, maintain and repair processes is becoming uneconomic. Therefore, how can an operator ensure that the risk of downtime is managed or minimized in the most effective way?
Much has been written about the advantages of the Internet of Things (IoT), Industry 4.0 and other initiatives, but are these approaches also relevant to addressing this risk to operations? The answer is a qualified ‘yes’. While IoT and Industry 4.0 approaches have already been shown to optimize logistics, process flows, inventory and the supply chain, the application of appropriate and cost effective sensors, process monitoring and analytics can also be used to:
- establish the normal behavior and range of settings and operating conditions for a process;
- provide early warning of drift and out-of-tolerance situations before process yield drops or a machine breaks down; and
- detect the emergence of equipment faults and identify their root cause
In other words, a so-called ‘expert system’ can be realized which captures normal operating conditions. This supports the operator in both remedying faults before the effects are felt, and in returning the process to operation quickly and reliably.
To allow this type of approach to work and to develop a solution for a manufacturing process, a scientifically rigorous approach is required to gather data on a process. The important parameters to monitor should be identified as should any irrelevant parameters. The interrelationships between process configuration and performance is critically important both to define a baseline (i.e. initial) setup and in fault diagnosis. This enables the failure mode to be understood and the repair, replacement, adjustment required to be specified.
A typical sequence of events might therefore be:
- Familiarization with the manufacturing process to gain an understanding of the parameters and settings to be monitored.
- Performing a monitoring exercise over several process cycles (typically days/weeks); monitoring a large number of parameters (including those which may appear irrelevant), using many temporarily applied sensors. Ideally, this should be performed during a range of process conditions from start-up to shutdown, changeover, and fault conditions. At this stage, the more data that is gathered, the better.
- Analyzing this data to identify the critical control parameters, and their effect on productivity, performance and product quality. It can also include identifying normal running conditions such as acoustic signatures, motor currents, flow rates etc. At this stage, unforeseen and even surprising relationships between sensors and process outputs may emerge; this is very much a learning phase.
- Using this analysis, define a realistically sized and cost effective set of sensors required to monitor the process for both performance and equipment condition.
- Developing a software model defining normal running conditions to enable future identification of out-of-tolerance situations.
- Designing a cost effective sensor and software system that can be fitted or retrofitted on each production machine. These can then continuously monitor the process and identify process drift, and in the longer term can include adjusting process settings to account for this.
All of this work requires the involvement of process experts, but when deployed successfully on production machinery, there are potentially a number of advantages:
- Comparisons can be made between many machines of the same type that reveal ‘outliers’; machines that behave differently to the remainder of the population.
- With the appropriate sensors deployed, the data collected can provide useful insight into the evolution of a fault — for example, precursor events can support rapid diagnosis and resolution.
- A baseline setup can be established for each machine so that the operator can quickly return the machine to production.
There is a direct analogy with the maintenance/fault-finding approach used in today’s cars where the Engine Control Unit (ECU) illuminates the engine warning light and provides an error code so that the technician knows where the fault lies and can make a rapid repair without the need to refer to an expert.
Operators will always require a degree of in-house process expertise. However, the application of appropriate sensing and machine learning does offer the very real prospect of distributing and automating the expertise currently residing in senior technical staff so that a business can reduce the risk of downtime, becoming less reliant on this increasingly rare and costly resource. Ultimately, this capturing, analysis and distribution of know-how holds significant promise for increasing business resilience through the reduction of the risk of extended downtime due to process failure.
Andrew Strong is Associate Director of Oil & Gas business at Cambridge Consultants.