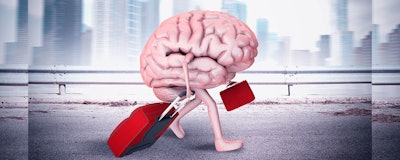
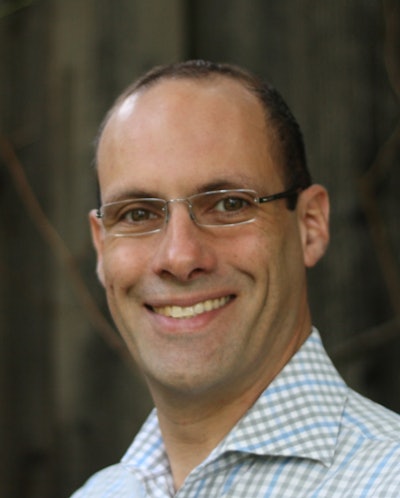
An estimated 10,000 baby boomers turn 65 years old each day, many of whom work in manufacturing-related positions. In industries that rely on the experience of the people who actually run the machines to maintain and sustain operations, a retiring workforce means retiring knowledge. So how do we transfer decades of accumulated know-how, or tribal knowledge, from the retiring generation of technicians and engineers to new hires entering the field?
Today, technology known as APM (asset performance management) helps operators catalog critical processes not only for the operations of today, but also for the next generation of employees. By collecting data automatically from sensors on plant machinery, APM can predict equipment failures and help operators prescribe a solution to a pending failure in real-time. How, when, where and why this is done, is captured by APM so the next time this issue occurs, the learning curve is shorter. By addressing the tribal knowledge problem, APM is transforming the siloed work culture and processes that helped create the problem in the first place.
The Root of the Problem
Before APM, operators didnβt worry about logging data entries consistently, and often classified equipment status differently. They also didnβt prioritize process documentation because the experienced engineers and operators performing them worked alongside colleagues who understood them well and were expected to be there for years to come. Fast forward to today and those same operators now find themselves in difficult situations given changing technology and industry regulations. And, those experienced employees are now βtrainingβ younger, more digital-savvy operators who donβt have the same βfeelβ for the equipment.
As the foundation of the manufacturing industry nears retirement, organizations must overcome knowledge loss. The knowledge drain can be so severe that many companies have opted to hire consultants to interview outgoing employees and glean valuable information before itβs too late. This process, however, is neither comprehensive nor cost effective. The difference between what a person says in an interview and what they actually do in the field can be immense.
Enter APM
APM helps manufacturers capture workersβ actions and processes directly. Simply defined, APM is a breed of software that draws analytics directly from machines and enterprise asset management systems to create a sophisticated framework for how and when to do maintenance. APM solutions pull this data in real-time, analyzing it and presenting it in a simple, easy-to-use dashboard that does not require a data scientist to operate. Rather than pulling technicians out of the field for days at a time to pick their brains, workers simply log their actions into APM, which automatically tracks the results.
Machine learning algorithms within APM systems further help translate years of misclassified information and lazy data entries into consistent, comprehensive records for an accurate view of equipment health. One manufacturing organizationβs reliability team tried to determine how often their assets failed and prioritize the list, but they ran into a situation where they realized that the breakdown indicator field in their Computerized Maintenance Management Systems (CMMS) was rarely populated, making it difficult to determine whether the asset actually failed. As a result, the average failure rate calculated using the raw data was 16,000 months. After applying machine learning algorithms with APM, the average failure rate was recalculated to be just 14 months. Several incorrectly marked work orders were corrected, and engineers working on these assets were able to develop a more accurate maintenance strategy.
By helping operators catalog their processes for future workers, APM technology greatly improves plant efficiency and reduces downtime. Given that on average, manufacturing plants lose an estimated $22,000 per minute of downtime, the need to streamline operations is as critical as ever for todayβs manufacturers. From an annual perspective, manufacturers experience an average of 350 hours of downtime each year, which translates to $462 million in lost revenue from downed equipment. The ability to eliminate the lack of knowledge that causes this downtime is critical to the future success of manufacturers. Without APM solutions, they run the risk of incurring detrimental costs at an ever increasing rate.
Looking Forward
Fundamentally, the tribal knowledge problem boils down to a lack of system integration and a lack of foresight by manufacturers. While companies are embracing new technology at a historical rate, others are falling behind due to their failure to recognize the rapid disappearance of their aging workforce. APM enables organizations to capture and catalog critical knowledge through a single, company-wide system that facilitates and standardizes data traceability and access. Whether manufacturers like it or not, less reliance on human knowledge is the future of manufacturing as we know it. By no means will technology render humans obsolete in the industry. Humans will always be needed to make sense of the big data that these new solutions create. There is simply a greater need for real-time solutions to problems that once devastated manufacturing plants. APM answers the call.
David Ovadia is a marketing and product management executive at GE Digital.