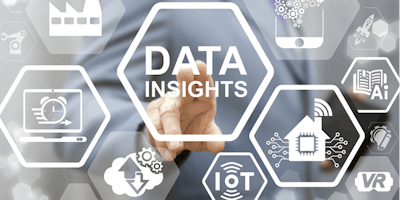
Bsquare Corporation
Manufacturing Page 1 © 2017 Bsquare Corp.
The discrete manufacturing sector is highly competitive and price sensitive, requiring
every machine, production line, and employee to contribute to achieving optimal yields,
high operational efficiencies, and cost controls.
Many manufacturing executives view advanced digital transformation technologies as
a critical path to competitiveness—especially predictive analytics, Industrial Internet
of Things (IIoT), and “smart” products and factories built on Industrial Revolution
4.0 (“Industry 4.0”)1. As of 2015, a full third of industry executives already rated their
company as “advanced” in factory digital transformation, and nearly 70 percent of
them expect to be at that level by 20202.
Despite this enthusiastic adoption of IIoT-equipped factory equipment, however,
only 3 percent3 of U.S. manufacturers are fully utilizing the invaluable operational
data they collect—data that holds the secret to improving output yields and quality,
predicting and avoiding plant-stopping failures, managing costs and resource usage,
and optimizing production lines in real time.
IIoT technologies such as advanced cloud-based data analytics, machine learning, and
predictive reasoning can assist manufacturers in transforming the rich operational
data they already collect into meaningful business information that helps them stay
productive and competitive.
1 Deloitte, “2016 Global Manufacturing Competitiveness Index (GMCI) Report,” p. 1.
2 PwC, “2016 Global Industry 4.0 Survey, “Industry 4.0: Building your digital enterprise,” April 2016, p. 4.
3 BGC, “Time to Accelerate in the Race Toward Industry 4.0,” May 19, 2016, chapter 2.
IIoT in Manufacturing
Transforming Factory Data
into Actionable Business Insights
IIoT in Manufacturing Page 2© 2017 Bsquare Corp.
Changes and Challenges for Discrete Manufacturing
The discrete manufacturing sector is undergoing one of the most transformative and
challenging business periods in its history—a fourth Industrial Revolution (“Industry
4.0”). This next phase in factory automation is driven by many new forms of digital
technology, including machine connectivity and data collection; data analytics and
business-intelligence capabilities; touch interfaces and augmented-reality systems;
and data-driven automation of complex rules and orchestration.
Companies that build cloud-connected assembly-line equipment, and the
manufacturers that use it, already employ a high degree of digital technology in
their mechanical, hydraulic, pneumatic, electronic, and computerized devices and
systems. Indeed, manufacturing is a leading industry for data collection—gathering
an estimated two exabytes of operational data annually4. Meanwhile, the price of
Internet of Things (IoT) technology, already on the decline since 2015,
is projected to drop 50 percent by 20205, and embedded sensors
are becoming standard features on new equipment. But while
35 percent of U.S. manufacturers are currently collecting
equipment data6, only 3 percent7 have taken steps to fully
exploit that information to improve their operations.
Advanced as factories have become, manufacturers
are still hard-pressed to remain viable and competitive.
Operations managers must consistently meet
performance, budget, and output forecasts, while also
ensuring product consistency, quality, and compliance. Plant
engineers must keep production lines operating, maintained,
and optimized, while also staying ready to alter production quickly
in support of new business priorities and revenue opportunities.
Meeting these objectives requires continuous visibility into the behavior and
condition of thousands of critical points across the factory floor. And such visibility
is already waiting within the raw operational data streaming from equipment,
collected from various systems, and stored throughout the business. The biggest
challenge is that most datasets are so massive and complex, they defy human
analysis. Companies simply lack the in-house expertise to dig through and extract
the right kind of knowledge to help them make smart, data-informed decisions the
moment they are needed.
4 Martin Baily and James Manyika, “Is Manufacturing ‘Cool’ Again?,” January 21, 2013.
5 McKinsey & Company, “Industry 4.0: How to Navigate Digitization of the Manufacturing Sector,” 2015, p. 12.
6 PwC and Zpryme survey and analysis, “2014 Disruptive Manufacturing Innovations Survey,” conducted February 2014,
cited by PwC
7 BGC, “Time to Accelerate in the Race Toward Industry 4.0,” May 19, 2016, chapter 2.
81%
of IoT adopters believe IoT
can only deliver real value
if they effectively use the
data it generates8
8 Vodafone, “Executive Summary:
Vodafone IoT Barometer 2016,” p.3.
IIoT in Manufacturing Page 3© 2017 Bsquare Corp.
Rather than leave this extraordinary opportunity for business improvement on
the factory floor, companies can use the power of Industrial IoT (IIoT) solutions to
convert their real-time, historical, and other systems data into meaningful business
knowledge to increase factory output, improve efficiency, and stay competitive.
Benefits of IIoT and Factory Data Analytics
Manufacturers regularly collect vast amounts of data from
thousands of sensors built into motors, conveyor systems,
5-axis machines, and other physical assets throughout
their factories. This data is typically used for equipment
monitoring and alerts, but that only scratches the surface of
IIoT’s potential to enhance production yield, quality, costs,
and compliance.
IIoT solutions can aggregate a company’s full repository
of real-time and historical data and apply advanced
analytics, predictive reasoning, and machine learning.
This sophisticated, automatic process unlocks previously
unknown operational insights to help manufacturers achieve
the “smart” digital manufacturing that Industry 4.0 is all
about.
Data analytics can, for example, help operators adjust real-
time production variables for greater yield and product
quality; monitor and maintain equipment health to avoid failures and lower servicing
costs; and optimize production lines as operational conditions change.
Opportunities to gain factory-wide insight and automate resource management
are available by incorporating historical operational data, as well as data
from other related systems, such as line-level programmable
logic controllers (PLCs), human-machine interfaces
(HMIs), manufacturing
execution systems (MESs),
enterprise manufacturing
intelligence (EMI) software,
parts inventories, and
enterprise resource planning
(ERP) systems.
85%+
estimated demand forecasting accuracy with
advanced analytics9
9 McKinsey & Company, “Industry 4.0: How to Navigate
Digitization of the Manufacturing Sector,” 2015, p.26
increased efficiency
Of organizations that use smart
manufacturing (Industry 4.0/IIoT)
experienced fewer
product defects
increased customer
satisfaction10
10 American Society for Quality, “Manufacturing Outlook
Survey 2014,” December 17, 2013, p. 2.
82%
49%
45%
IIoT in Manufacturing Page 4© 2017 Bsquare Corp.
Once appropriately analyzed and acted upon, a company’s streaming and stored
data become vital business assets in their own right—enabling production
improvements, cost savings, and smarter resource allocation. For instance,
manufacturers can use IIoT-based data analysis to:
nn Establish condition-based maintenance schedules to reduce unplanned
equipment downtime, better manage servicing costs, optimize production, and
extend the useful life of equipment
nn Use rules-based automation and remote control access to maximize yield
while also maintaining quality, prolonging equipment lifespans, and remaining
compliant
nn Automate and connect every corner of the factory floor to optimize processes
and material flow for more precise planning, just-in-time manufacturing, and
workplace safety
nn Make machines more autonomous, such as automated guided vehicles (AGVs)
and industrial/collaborative robots, to reduce the workload of IT, operations,
and engineering staff
nn Find the core determinants of production and workflow performance, then take
action to continually improve them
And these are not the only applications of IIoT that can significantly improve
financial outcomes. A PWC survey of industrial sectors, for example, projects that
10 percent of all companies that digitally transform their factories, and 27 percent
of “first movers” that do so, will simultaneously achieve more than 30% increased
revenue and more than 30 percent reduced costs by 202011.
11 PwC, 2016 Global Industry 4.0 Survey, “Industry 4.0: Building your digital enterprise,” April 2016, p. 12.
IIoT in Manufacturing Page 5 © 2017 Bsquare Corp.
Three Key Uses of Factory Data
IIoT technology delivers direct benefits in three critical areas for discrete
manufacturing companies: condition-based maintenance, asset optimization, and
smart asset utilization.
Condition-Based Maintenance
Any form of downtime affects manufacturing capacity.
Failure of even the smallest factory component can halt
production, delay delivery, impact revenue forecasts,
and create havoc along a manufacturer’s supply chain.
Every hour spent redistributing workloads to other lines,
diagnosing root causes, and making repairs adds to the
financial burden. The immediate and substantial benefits of
avoiding such issues are why companies invest in safeguards
like preventative maintenance. However, manufacturers
have historically employed maintenance procedures based
on fixed intervals, like time or usage, which fail to account
for a machine’s actual condition and operational fitness.
Often, this leads to costly over- and under-servicing,
which heightens potential risk of early failures, unplanned
downtime, expensive emergency repairs, shorter equipment
lifespans, and unnecessary maintenance costs.
A fundamental application of IIoT is the ability to harness real-time and historical
data from across a manufacturing plant to construct (using machine learning) highly
accurate digital models of the plant’s physical components. IIoT software then
leverages data analytics to detect changes, predict potential problems, send alerts,
identify probable causes, and present technicians with likely solutions based on
similar previous occurrences.
Operations managers and engineers can intelligently accelerate or delay repairs
according to actual asset condition. This can extend the life of harder-working
equipment and avoid the unnecessary cost of over-servicing. Using data analytics
to prescribe optimal maintenance intervals also contributes to smoother operations
and reduces, or even eliminates, unplanned downtime.
Asset Optimization
Actual performance of critical factory equipment can vary from unit to unit,
production line to production line, and factory to factory. Similar machines can
reduction in overall
maintenance costs
savings in scheduled
repair costs
IIoT-based predictive asset
maintenance leads to
fewer breakdowns12
12 Accenture, “Smart Production, Finding a Way Forward:
How Manufacturers Can Make the Most of the Industrial
Internet of Things,” 2015, pg. 7.
up to 12%
up to 30%
up to 70%
IIoT in Manufacturing Page 6© 2017 Bsquare Corp.
exhibit substantial variance in efficiency, output quality, and yield due to a variety
of factors. Continuously streaming data conveys each machine’s inner workings
in real time, but it is beyond most human capabilities to analyze it quickly and
accurately enough to detect equipment inefficiencies, assess variations
in production capacity, and adjust to achieve optimal operational
performance almost on the fly.
Applying machine learning and more advanced data
analytics to digital models, IIoT solutions can define
the behavior and operating parameters of optimally
performing factory equipment. This characterization
includes equipment settings, environmental conditions,
calibration, service intervals, configurations, and other
variables. The “optimized profile” can then be applied
across similar assets to improve underperforming
equipment.
Performance adjustments can be made remotely by engineers
or automatically by the system—such as to correct for quality
anomalies, account for ambient humidity, or temporarily reduce output speeds
if a machine’s operating temperature exceeds specific thresholds.
This optimization process—which is actually a continuous, ongoing aspect of IIoT—
becomes more intelligent over time and helps ensure equipment continues to run as
expected and as cost-efficiently as possible.
Smart Asset Utilization
Without full visibility into all factory operations—as well as the ability to adjust
systems automatically and remotely—manufacturers cannot easily define and
implement production workflows that will lower costs while improving efficiencies
and quality. Manual, unit-by-unit examination and reporting of machine status and
compliance-related data limits factory-wide view, and has inherent time delays and
potential for error. Managers have difficulty projecting inventory needs as well as
coordinating parts and materials orders for just-in-time production.
IIoT systems, using rules-based automation and advanced data analytics, can
consolidate utilization data from all connected equipment into a single dashboard
that is viewable from anywhere. Moreover, they can integrate factory-floor data
with that of other sources, such as line-level PLCs, HMI systems, MES, EMI, parts
inventories, and ERP systems. Rules-based logic applied to this aggregated data
can automate complex, conditional actions (e.g., inventory adjustment requests),
up to 25%
estimated increase in
production volume from big
data/advanced analytics13
13 McKinsey & Company, “Industry
4.0: How to Navigate Digitization of
the Manufacturing Sector,” 2015, p. 11.
IIoT in Manufacturing Page 7© 2017 Bsquare Corp.
enforce business policies (e.g., operator hours, safety parameters), and ensure
compliance in regulated industries (e.g., emissions, disposal).
The use of data to observe, understand, and automate equipment and resource
utilization enables companies to oversee all manufacturing and assembly activities
with the aim of optimizing production, ensuring workplace safety, and maintaining
compliance.
Key Uses of IIoT in Discrete Manufacturing
Connect physical assets for:
• Data collection and transmission to the network, cloud-based databases, and applications
• Filtering, prioritization, and pre-processing of data
• Local business rules and actions of assets
Monitor asset data to:
• Apply logic to real-time data streams from physical assets both locally and remotely
• Run complex event detection in real-time
• Establish a distributed rules engine for anomalous conditions
Predict conditions to:
• Best identify circumstances that frequently precede failures and orchestrate complex actions
• Integrate into enterprise systems to prevent predictable failures
• Schedule preventative maintenance at optimal intervals to eliminate costly unplanned downtime
Automate operations with:
• Machine learning that optimizes equipment efficiency and lowers the cost of failures
• Predictive modeling that plans for anomalous conditions detected by the monitor function
• Automatic rules generation to perform actions such as adjusting heat treatment time and tem-
perature to prevent product defects or improve yield
Optimize equipment performance through:
• Digital asset modeling that can benchmark operational parameters of high performing equipment
• Performance optimization that applies the parameters used for the best performing equipment
• Prescriptive remediation that can improve abilities of less than optimal assets
IIoT in Manufacturing Page 8© 2017 Bsquare Corp.
The Bottom Line for Discrete Manufacturers
The emergence of Industry 4.0 and IIoT is
transforming manufacturing yields and operational
efficiency around the world. IIoT, in particular,
holds the key to helping manufacturers transform
the data they already collect into the business
knowledge they need to increase output, manage
costs, and improve productivity.
Benefits of enhanced visibility into all operational
and mechanical data include the ability to:
nn Ensure the execution of manufacturing strategy
nn Develop consulting solutions, optimize workflows, and drive improvement
initiatives
nn Manage capital investments and high-level planning
nn Consistently meet KPIs (e.g., performance, budget, forecasts)
nn Promote and deploy continuous improvement initiatives, techniques, and
practices
nn Analyze operational data and make real-time adjustments as needed
To find out more about how your organization can best embrace IIoT for maximum
impact, please email [email protected] or call 425-519-5900.
About Bsquare
For over two decades, Bsquare has helped its customers extract business value from
a broad array of assets by making them intelligent, connecting them, and using data
collected from them to improve business outcomes. Bsquare software solutions have
been deployed by a wide variety of enterprises to create business-focused Internet of
Things (IoT) systems that can more effectively monitor assets, analyze data, predict
events, automate processes and, in general, optimize business outcomes. Bsquare
couples innovative software with advanced professional services that can help
organizations of all types make IoT a business reality.
© 2017 Bsquare Corporation. Bsquare and DataV are a registered trademarks of BSQUARE Corporation in the U.S. and other countries. Other names and brands
herein may be trademarks of others. Phone: 888.820.4500
28.5%
average year-over-year revenue increase in 2014
for industrial manufacturers using IoT solutions14
13 Tata Consultancy Services, “Internet of Things: The Complete
Reimaginative Force,” TCS Global Trend Study, July 2015, p. 56.