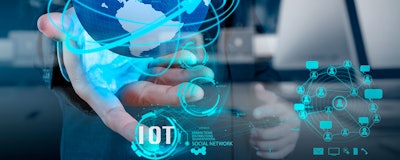
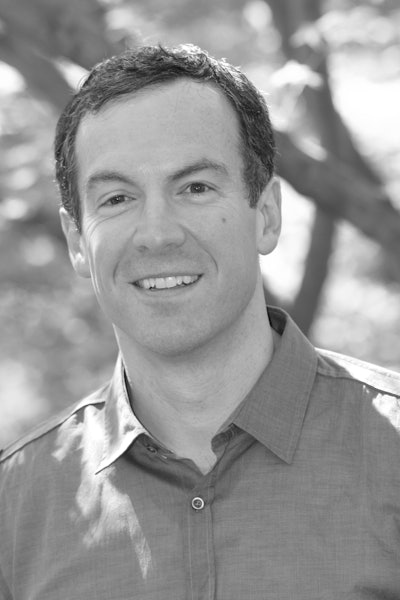
In December, 2015 Accenture interviewed executives across several major industrial sectors about their strategies for creating a more connected workforce. At the time, the overwhelming sentiment stated that smart devices and IoT-enabled assets would come together to enable whole new levels of operational performance and safety, but that most manufacturers still lacked the confidence to implement such a program successfully.
Since that time, there’s been a significant awakening to just how important edge computing is to a more connected workforce. So much of the discourse has revolved around the concept of products, people, processes and services communicating with each other over the Internet that the importance of direct asset-to-asset and asset-to-person communication has somewhat been lost in the jumble.
To that end, 2017 probably marked the end of the hype cycle for the IIoT. From here, there’s no more excuses. We need to look no further than the fact that AWS — the veritable progenitor of mass market cloud computing as we know it — acknowledged with its launch of Greengrass that data analysis capabilities had to move closer to the edge. The value of the IIoT in distributed environments was unlocked once and for all by paving the way for asset-generated data consumable by on-the-spot humans in near real-time, to make faster decisions, improve asset utilization, minimize downtime and simply gain a more granular level of control over the processes. To do that, these assets need to be able to share information without the assistance of a cloud-based intermediary. For example, the safety equipment in an airliner needs to talk directly to the pre-flight inspection crew. Or, the hydraulic control valve on an industrial robot needs to tell a factory technician when the hoses were last serviced or replaced.
The real key for moving from discussions about what’s possible, however, to actually doing what’s practical is understanding the need for a simple, safe, efficient and cost-effective approach to edge infrastructure. Luckily, this answer is already right under our nose. It is impossible to understate how large the opportunity is to embrace both iOS and Android as dominant OS options for edge system equipment. Over the last decade, society has come to a place where we walk around with a tablet or mobile phone that has more computing power and connectivity as something that would have been deemed a ‘supercomputer’ only 15 years ago. Consequently, virtually everyone has the power in their pocket to simply walk up to an operational asset and initiate a smart interaction with it. You can query the asset’s lifecycle history, download information about its installation or write dynamic condition updates to it. Essentially, 2018 will see modern consumer devices gain their due place as the missing link to analytics at the edge.
Once an asset-heavy organization makes this mental leap, assets can start to be seen for more than their pure operational purposes. They are more than endpoints that flow information to the cloud. They are repositories for information that can be retrieved and updated at any given time throughout their lifespan, by any member of the workforce with a smartphone-based reader and proper security credentials. And it requires zero cloud connectivity of any kind.
It is here that edge analytics becomes an antidote to the anticipated — and pragmatically limiting — deluge of unstructured IoT data. We know that edge devices are getting smarter and generating minute-by-minute streams of data, but with IOS and Android devices in place to intercept and interpret the most prescient of this data, only a subset must now be sent to the cloud for more resource-intensive processing and interpretation. There’s a streamlining effect on the data, while the path to vital decisions takes a less circuitous route.
Herein lies a subtle, but nonetheless critical factor for manufacturers staring down a skills gap crisis. For workers new to the job, edge analytics provides a built-in means toward job training and skill development. If an asset has been embedded with the right data, it can actually dictate its maintenance or operating instructions to employees as they interact with it. The asset literally becomes the informed trainer.
At the other end of the spectrum, experienced workers now have a way to transfer “tribal knowledge” they’ve accumulated over the years more broadly across the organization. In fact, edge data allows these workers to understand that technology is not here to replace them. On the contrary, they are empowered to show people just how smart they are by embedding their personal tricks of the trade into the assets they work with every day, to be absorbed by the next colleague down the line.
Functionally, edge computing shapes up as a distributed model that mimics the chip-hardware/bus-OS stack that is at the heart of consumer device computing. Without a wide scale perspective shift that envisions an OS-layer build upon iOS and Android as the node for direct interaction with static assets, the ramp of hope for digital transformation may not be as steep as pundits believe.
Timothy Butler is the founder and chief executive officer of Tego.