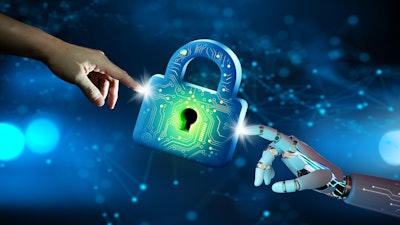
Industrial control systems (ICS) have become both the backbone of modern manufacturing and a prime target for sophisticated cyber adversaries. Traditional, signature-based defenses designed for yesterday’s threats are increasingly outmatched by novel attack techniques, insider threats, and AI‑driven malware.
Against this backdrop, artificial intelligence (AI) and machine learning (ML) are emerging as pivotal tools for mitigating threats to industrial cybersecurity. Let’s explore the evolving threat landscape, the shortcomings of legacy approaches, the revolutionary potential of AI/ML, real‑world applications in manufacturing, and the practical challenges organizations must navigate to build resilient, future‑proof ICS security architectures.
The Escalating Threat Landscape for Manufacturing
The digital transformation of manufacturing has unlocked massive productivity gains, but it’s also invited a surge in cyber adversaries probing for weaknesses. As plants connect legacy interfaces to corporate networks, adversaries can exploit these vulnerabilities to launch targeted attacks. 5G deployments and IIoT (Industrial Internet of Things) sensor proliferation further magnify risk, as each endpoint becomes a potential foothold for attackers. All of this not only obscures your threat visibility but also undermines traditional perimeter defenses, allowing malicious actors to navigate ICS protocols undetected.
State‑sponsored groups and cybercriminal syndicates alike have turned their attention to ICS, recognizing the potential to inflict physical damage or extort organizations via ransomware. The Sandworm group has leveraged custom ICS malware to strike energy grids and manufacturing plants. Furthermore, the Colonial Pipeline attack underscored how ransomware can paralyze critical supply chains, forcing a multi‑day shutdown of one of the East Coast’s largest fuel distributors and triggering widespread economic disruption.
Meanwhile, industrial sectors face a ransomware onslaught, with a recent Dragos report finding that manufacturing entities accounted for 69 percent of all ICS‑related ransomware incidents, a staggering 87 percent surge over the previous year. As a result, industrial insurance costs are skyrocketing and manufacturing businesses are having to invest heavily in cybersecurity.
Limitations of Traditional Cybersecurity in the ICS
Conventional IT security can fall short in ICS contexts for several reasons. Whereas IT defenses prioritize data confidentiality and network integrity, ICS security must place equal weight on system availability and the physical safety of processes and personnel.
Many ICS devices operate on proprietary or outdated operating systems that can’t support modern endpoint protection, and their decades‑long lifecycles make frequent patching impractical. Moreover, embedded controllers often lack the computational resources for encryption or advanced access controls, leaving your critical assets exposed.
Signature‑based detection, the workhorse of traditional security, only flags threats that match known patterns, rendering it ineffective against zero‑day exploits, polymorphic malware, or adversaries who deliberately craft novel payloads for OT environments. Asset inventories and vulnerability assessments are typically maintained by hand, a time‑consuming process that quickly falls out of sync in sprawling factory networks.
Effective ICS defense also demands specialized domain knowledge, from understanding SCADA communications to compliance with sector‑specific safety regulations, skills that are in chronically short supply. Attempting to bolt on IT‑centric responses, such as network segmentation without process awareness, can inadvertently disrupt control loops or even trip safety interlocks, risking unplanned shutdowns or physical harm.
Revolutionizing ICS Cyber Defense
AI and ML are rewriting the playbook for ICS security, bringing capabilities that far exceed manual and rule‑based approaches. ML models can be trained on historical network flows, sensor outputs, and control commands to establish “fingerprints” of normal operations for every device in a plant.
When real‑time telemetry deviates from these learned baselines, even in subtle ways undetectable by human analysts, AI platforms can flag anomalies within milliseconds. Unsupervised learning techniques can uncover previously unseen threat vectors, while reinforcement learning can adapt detection thresholds on the fly to minimize false positives.
Beyond detection, AI systems excel at correlation. They ingest logs from firewalls, endpoint sensors, PLC telemetry, and even weather feeds to generate a unified threat portrait across both IT and OT domains. This holistic visibility is essential when adversaries leverage blended tactics, such as phishing on the IT side to commandeer credentials, then jumping to OT networks to manipulate control logic.
Automated incident response modules can isolate compromised segments, quarantine suspect endpoints, and even execute safe shutdown procedures, all under the supervision of human operators to ensure minimal production disruption.
Predictive analytics powered by AI can forecast potential attack scenarios by mining threat intelligence feeds, past incident data, and sector‑wide vulnerability trends, enabling security teams to shore up defenses proactively. Meanwhile, risk‑based prioritization engines can sift through thousands of vulnerabilities, weighting each by asset criticality and potential impact on process safety, so you know exactly which patches or mitigations demand immediate attention.
AI‑driven asset discovery continually profiles both known and rogue devices, maintaining accurate inventories that feed segmentation policies and compliance audits. AI can bridge entrenched silos by unifying IT and OT under a common analytics framework to foster collaboration and elevating defensive postures across the entire enterprise. Crucially, these platforms alleviate talent shortages by automating routine analyses, allowing scarce security engineers to focus on strategy and high‑fidelity alerts.
Manufacturers around the globe are ditching static firewalls for AI-powered, learning-driven defenses that adapt to threats on the fly. Take DriveAuto Technologies in Detroit, for example: they integrated real‑time AI malware detection across their assembly lines, so their Cybersecurity Management Center can spot and quarantine suspicious activity in milliseconds, without any unplanned production stoppages. Supervised and unsupervised learning allows DriveAuto’s system to build dynamic baselines for what ‘normal’ looks like on every device, catching weird behaviors that would slip past old‑school signature tools.
Another global manufacturer leaned on Cylance’s AI‑powered endpoint protection for their legacy PLCs and HMIs, using pre‑execution ML to predict and block a zero‑day payload aimed at crippling control devices. Because the AI nipped the malware in the bud, they kept running full tilt. No emergency stops, no costly downtime.
The Challenges of Implementing AI in ICS Security
Despite the promise, AI adoption in ICS environments isn’t just plug‑and‑play. High‑quality, contextualized data streams are the lifeblood of ML models, yet many facilities can struggle to normalize and label data from legacy devices or proprietary protocols.
A shortage of professionals fluent in both OT operations and AI techniques exacerbates the skills gap, making it hard to build, tune, and interpret ML systems. Moreover, adversarial actors may probe AI models themselves by feeding poisoned data to elicit false negatives or crafting inputs designed to bypass anomaly detectors.
So you can mitigate these risks, organizations should adopt a phased integration plan: start with pilot projects on non‑critical lines, validate your cybersecurity model performance with human‑in‑the‑loop oversight, and gradually scale to cover mission‑critical assets. It’s equally important to secure the AI infrastructure: hardening training environments, encrypting model weights, and employing digital watermarking to detect tampering.
To guard against adversarial manipulation, you’ll need robust monitoring of AI outputs, periodic model retraining, and red‑team exercises to help ensure detection fidelity and guard against adversarial manipulation. The convergence of IT and OT has thrust ICS security into the spotlight, revealing the inadequacy of legacy defenses against increasingly sophisticated threats. But AI and ML can respond with capabilities that were unimaginable a decade ago.
For manufacturing leaders and security professionals, the imperative is clear: assess your current ICS security posture, invest in AI/ML education and pilots, and pursue unified, data‑driven platforms that can adapt as threats evolve. You’ll not only defend against today’s attacks, you’ll also build the operational resilience needed to stay one step ahead of tomorrow’s adversaries.