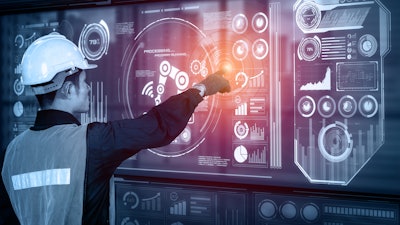
Across industries, companies are feeling pressure to digitally transform, which often gets misconstrued with simply generating an unprecedented amount of data. However, for manufacturers, a surprising truth exists: they are already sitting on a treasure trove of data. The crucial next step lies not in generating more data, but in making use of what they already have to drive additional value.
By learning how to utilize data effectively, manufacturers can gain efficiencies in increments of just one to five percent. Though these gains seem small, they can be substantial for asset-heavy industries. For instance, eliminating just two days of turnaround time translates to two additional days of production, potentially resulting in millions in additional revenue.
This also reduces energy consumption, minimizes wear and tear, and places less stress on equipment. Despite data’s significant potential, only three percent of manufacturing executives fully trust the data they use to make critical decisions. I believe this lack of trust stems from the industrial data problem.
Defining the Industrial Data Problem
A typical industrial facility routinely generates over 100,000 data points across more than 50 applications. Which makes it unsurprising that 56 percent of executives selected data consolidation as the top capability that would improve their trust in data, followed by contextualization and mapping relationships across datasets (52 percent). For many manufacturers, the data exists – but it lives in silos, comes in a multitude of formats lacking a common, universal language, and is often used to solve just one or two narrow business problems.
In fact, it generally takes more time to pull data from the various different sources than it does to analyze and understand what it’s saying. To begin using data to make businesses more efficient, manufacturers must first identify what kind of data they’re looking for, where to find it and what it’s telling them.
Manufacturing data can be found across various systems like historians, IoT platforms, MES, ERP, CMMS, PLM, engineering/design data and more, but again, the fragmentation limits its usefulness. While sourcing individual data points can provide some value and solve specific problems, true business-transforming value comes from first pulling the data into one place. The next step is making sense of the data and using it to identify trends, or, contextualizing it.
Contextualization is the process of identifying and representing relationships between data to mirror the relationships that exist between data elements in the physical world. The result is a richer data model that is greater than the sum of its source systems. Contextualization paints a fuller picture of the business and makes the data actionable and easier to understand.
Once the data is consolidated into a single repository and contextualized, it becomes a powerful tool for driving immediate impact. For manufacturers who don’t know where to begin, there are five key operational areas I recommend as a starting point. These areas are typically bogged down by manual processes and present the greatest opportunities for ROI. This includes:
- Shutdown time. A key performance indicator for industrial data operations is minimizing downtime to maximize production time. Contextualized data can be used to find opportunities to conduct simultaneous operations during shutdowns, delivering a 20 percent time savings. Data can also be used to better monitor equipment and reduce risks of failure.
- Real-time data efficiencies. Having access to real-time data is game-changing for organizations that have struggled with manually pulling data from various sources. In manufacturing, real-time data access enhances operational decision-making, therefore increasing site safety, predictive maintenance and machine performance. One of our customers used our industrial DataOps product to provide their insurer with live data which greatly reduced the insurance risk associated with their heavy equipment. Another used the data to support superior training of their machine learning model in production, which led to increased performance levels in production processes.
- Planned maintenance. Contextualized data that provides increased visibility into resources, workflows and component lifecycles allows SMEs to optimize planned maintenance. I’ve seen large asset downtime decrease from 34 percent to four percent, simply by utilizing these kinds of processes.
- Energy savings. Manufacturing currently accounts for 25 percent of the United States’ energy consumption, and with rising energy costs, that’s a significant financial burden on manufacturers as well. Contextualized data helps save energy by optimizing processes and facilitating predictive maintenance. Predictive maintenance uses a combination of sensors, contextualized data and machine learning to monitor equipment performance and identify signs of trouble before they evolve into full-blown failures. This allows for timely repairs and ensures malfunctioning equipment doesn’t continue operating. Process optimization is all about taking a critical look at manufacturing processes and identifying areas for improvement, with the goal being to eliminate inefficiencies that lead to wasted energy.
- Heavy machinery optimization. One of the biggest benefits that comes from contextualized data is the optimization of heavy machinery and industrial processes. The longer the asset is in production and operating effectively, the greater the ROI. Machine learning and real-time data can be used to optimize performances and keep production running longer.
By addressing the industrial data challenge, manufacturers can better understand and use their data to improve efficiency in key operational areas. This can help minimize shutdown times, optimize machinery use and reduce energy consumption. The path to digital transformation starts with utilizing the available data to enhance efficiency and reduce costs.
Laxmi Akkaraju is the Chief Customer Officer at Cognite