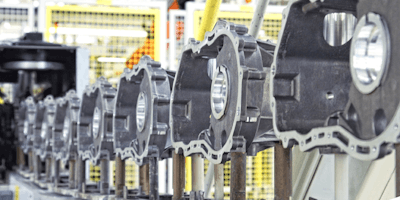
Pros
THE CRITICAL VARIABLE IN SERVICE PARTS PRICING:
UNDERSTANDING WILLINGNESS-TO-PAY
Unlock Your Data • Unleash Your Sales
WHITE PAPER
By Sean Duclaux
Director, Industry Marketing
OPTIMIZING PRICING FOR THE AUTO/EQUIPMENT PARTS INDUSTRYOPTIMIZING PRICING FOR THE AUTO/EQUIPMENT PARTS INDUSTRY
32
DETERMINING WILLINGNESS-TO-PAY WITHIN REACH OF
SERVICE PARTS COMAPNIES
Although revenue generated
from service parts factor
into a company’s profits,
few service parts suppliers
take full advantage of the
opportunity pricing offers.
When dealing with the pricing
complexities of thousands of parts,
broad cost-plus pricing strategies
are the industry norm, when in fact,
a customer’s willingness-to-pay is
independent of the production costs of
the service part.
The term “Willingness-to-Pay” (WTP) is
frequently used to describe the
spending limit of customers for products
they buy. As defined by Christoph
Breidert, an author and Ph.D. in
business and economics: (1)
Willingness-to-Pay is the highest price
a buyer (e.g., individual, corporation,
dealers, distribution channel, etc.) is
willing to accept to pay for some good
or service.
It is important to understand that all of
the various types of customers
within the service parts market are
encompassed in Dr. Breidert’s reference
to a buyer. Examples of different
customers in the service parts market
are the airlines that fly commercial
aircraft, a dealer franchise of a Detroit
Big 3 automaker, a company like FedEx
that owns a national fleet of trucks,
traditional parts distributors like NAPA,
or the many independent warehouse
distributors, jobbers, and installers
that purchase the part directly or from
a multi-tiered channel. The WTP will
vary greatly by the type of service
parts customer, their position along
the distribution chain, and the pricing
pressures or availability of the service
parts supply.
The concept holds promise for service
parts manufacturers and distributors
when trying to estimate the right price
for each of their products and markets.
If you knew each buyer’s precise WTP
for each product, you could simply
charge that price. The likely result would
be a huge increase in your profit and
market share.
MOVE FROM “COST-PLUS” TO VALUE PRICING
Many service parts companies still use cost-plus pricing techniques as a
general way to price their products. Example: price = cost plus 45 percent
of manufacturing cost. Once prices are set, the effectiveness of that
pricing is judged by changes in volume and the product’s P&L statement.
In contrast, prescriptive pricing software technology offers a scientific,
practical alternative that determines each buyer or buyer category’s WTP.
Prescriptive pricing software from PROS, for example, is able to
dynamically examine a history of transactions and adjust WTP as data
comes in. Operating at a highly granular level, buyer by buyer, product
by product, PROS makes a value-based pricing approach possible —
estimating the value that buyers actually put on products versus what they
might currently be paying. Such pricing insight is critical to ensuring that
manufacturers and distributors gain and sustain a competitive advantage
through value-based service parts pricing.
OPTIMIZING PRICING FOR THE AUTO/EQUIPMENT PARTS INDUSTRYOPTIMIZING PRICING FOR THE AUTO/EQUIPMENT PARTS INDUSTRY
32
The next logical question, then, is how
you can measure your buyers’ WTP. In
2006, researchers Breidert, Hahsler
and Reutterer (2) reviewed the current
methods for measuring WTP along
with others such as Nagle and Holden
(3). These authors categorize methods
for determining WTP into four key
approaches: experiments, direct
surveys, indirect surveys and market
data.
Common business techniques to
determine WTP, however, don’t apply
very well in the service parts marketing
environment. Experiments and surveys,
while appropriate in business to
consumer markets, don’t appear to
work well in service parts business-
to-business markets for a couple of
reasons. First, experiments are very
hard to implement through a sales
force based on commissions.
Second, the procurement departments
are unlikely to participate in a focus
group or give reliable answers to
questions such as, “At what price
point would you stop ordering this
product?” However, indirect
survey methods can be quite valuable
when working with buyers at your top
national accounts. With a focus on a
handful of key accounts and the core
products they buy, it is possible to gain
insights into their perceptions of the
value you provide. But, how do you
determine the best price to ask of
the other 98 percent of the target
accounts?
Most service parts manufacturers and
distributors can determine WTP only
by relying on market data; however,
the researchers cited here give little
guidance about how to actually
measure WTP with market data. Nagle
and Holden concede that
“. . . if a researcher has a lot of
historical data with enough price
variation in it, useful estimates of price
sensitivity are possible.” (3) Here’s
the good news for companies in
service parts industries:
And, the key to unlocking WTP can
be found in applying the science
of pricing to your own service parts
transactional market data. First,
however, you need to understand
some basic principles behind WTP.
Your transaction data has a wealth of
information that can help isolate and
identify your buyers’ WTP across your
product portfolio.
OPTIMIZING PRICING FOR THE AUTO/EQUIPMENT PARTS INDUSTRYOPTIMIZING PRICING FOR THE AUTO/EQUIPMENT PARTS INDUSTRY
54
SEGMENTATION AND NORMALIZATION:
START WITH YOUR OWN DATA
Service parts companies
typically possess plenty
of data around their own
products (cost, monthly
volumes, product hierarchy,
lifecycle stage, etc.), their
customers (geography, size,
industry, etc.) and their
transactions, invoices and
rebates (date, price, cost,
discount, sales person,
quantity, unit of measure,
etc.).
Do the supplier and customer
relationships in different geographies
have a different WTP? Does my
purchasing agent have a discount
expectation based on the quantity
ordered? Do distributors react
differently to a service part that costs
$4,000 than to a service part that costs
$20? Do jobbers notice price changes
equally across all brake components,
fluids and other maintenance items,
or are there individual parts within
each of these categories where a price
increase is noticed less?
The science of segmentation answers
these questions clearly and decisively
by using your existing service parts
sales data. The process involves
applying scientific algorithms, using
computer software to mine your
data and analyze all combinations
of attributes, to determine the key
variables that impact your customers’
WTP.
While there are numerous attributes
to consider (e.g., geography, OEM
vs. aftermarket, reman vs. new,
ecofriendly, etc.) determining WTP
can still be challenging given certain
regulatory and legal constraints.
Service part manufacturers, for
example, are bound by agreements
with their distributors — differentiated
pricing by dealer is strictly prohibited.
Rebates and volume discounting
may offer some opportunity to create
differentiation, but, in general, service
part suppliers that sell through a
dealer network must set a single
PRESCRIPTIVE PRICING BASED ON WTP PRODUCES RESULTS
Using extensive data on tens of thousands of products, PROS pricing
software was able to analyze and determine WTP for a multi-billion
dollar distributor. By automating cumbersome manual processes and
using statistical methods to segment its customer base into peer groups,
PROS provided scientifically generated target, floor and stretch (e.g.,
expert) pricing guidance. Guidance increased margins of low-performing
customers in each peer group, while ensuring that high performers
continued to be highly profitable.
The PROS pricing solution helped reduce “below floor prices” more
than 10 percent while increasing the number of invoices exceeding sales
targets more than 10 percent, resulting in a gross profit increase of more
than 230 basis points. Revenues increased by more than two percent in
individual segments.
OPTIMIZING PRICING FOR THE AUTO/EQUIPMENT PARTS INDUSTRYOPTIMIZING PRICING FOR THE AUTO/EQUIPMENT PARTS INDUSTRY
54
price per service part. Without price
differentiation, you may question the
possibility of accurately calculating
your customers’ WTP.
The solution to this challenge involves
more thoughtful analysis of your
product attributes.
Consider the following scenario: Part
Manufacturer A, an American-based
company, sells an environmentally
friendly brake pad made of recycled
materials. Not surprisingly, demand
for this product is highest among the
part manufacturer’s customers located
in environmentally sensitive areas
of the US, such as the west coast.
Therefore, the part can be described
in terms of its customer attributes;
we would say, for example, the brake
pad is “west coast centric.” Given this
information, Part Manufacturer A will
still set a single optimized price for
all customers, but it will do so based
on demand and profitability on the
west coast, allowing the company to
capitalize on revenues where it expects
its highest sales volume.
The concept of geographic centricity is
not limited to environmentally friendly
characteristics. Due to differing vehicle
sales volumes, brand preferences and
climates, you will find that most parts
become centric to one region or
another. And, centricity doesn’t have to
be limited to geography. Parts can be
“centric” in terms of other customer
characteristics, such as dealership size.
Therefore, even though many service
parts companies cannot price
differentiate across customers, they
can still use customer attributes
to describe how a part sells in the
marketplace and determine the
optimal price.
Where differentiation by customer
attributes is limited, pricing science
provides service parts companies with
the ability to differentiate by product
attributes, thereby allowing them to
increase their revenues and drive their
overall profitability. These factors make
targeted pricing a real challenge for
parts manufacturers and distributors,
but understanding your customers’
WTP as well as how your products
differentiate WTP in the market
can make a big difference in the
performance of your pricing decisions.
Moreover, it is important to remember
that segmentation alone does not
account for the dynamic nature of WTP
discussed earlier.
Normalization is the principle that
applies a time-based approach to
WTP that enhances the accuracy of
pricing products. Normalization works
by looking at external factors (date,
producer price index, inflation, etc.)
that vary over time and then adjusts
historical transactions appropriately.
Economists use this technique all the
time. For example, gold is at an all-
time high, but not when normalized
against history. The early 1980s holds
the price record when you adjust for
inflation (4).
The science of segmentation puts
different customers, channels, parts
and transaction environments in
separate WTP buckets. Normalization
changes historical data inside each
bucket to account for WTP changes
over time. But you must go another
step further by looking inside each
segment and determining the unique
WTP for each product through
distribution analysis.
OPTIMIZING PRICING FOR THE AUTO/EQUIPMENT PARTS INDUSTRYOPTIMIZING PRICING FOR THE AUTO/EQUIPMENT PARTS INDUSTRY
76
300
0
250
200
150
100
50
$1
.00
$1
.03
$1
.05
$1
.08
$1
.11
$1
.14
$1
.18
$1
.21
$1
.25
$1
.29
$1
.33
$1
.38
$1
.43
$1
.48
$1
.54
$1
.60
$1
.67
$1
.74
$1
.82
$1
.90
$2
.00
$2
.11
$2
.22
$2
.35
$2
.50
$2
.67
$2
.86
$3
.08
$3
.33
$3
.64
$4
.00
Actual Margin Data
Figure 1 - Pricing science can estimate the true Willingness-to-Pay even if loss data is unavailable.
This chart shows the price distribution of a service part based on win data.
OPTIMIZING PRICING FOR THE AUTO/EQUIPMENT PARTS INDUSTRYOPTIMIZING PRICING FOR THE AUTO/EQUIPMENT PARTS INDUSTRY
76
USING SALES TRANSACTION ANALYSIS TO ESTIMATE
WILLINGNESS-TO-PAY
Once you’ve segmented
and normalized your
purchasing data, the next
step is to examine the
distribution of prices within
each specific segment.
How you proceed from here depends
on the availability of loss data. If you
know those price points in a segment
at which your customers walk away and
at which they buy, a clear distribution of
WTP emerges. Research by Ferguson
and Agrawal (5) and Robert Phillips
(6) explains how to use loss data to
model win elasticity across a specific
customer segment. Unfortunately,
most service parts manufacturers and
distributors don’t have access to their
loss information.
Even if you could mine the data from
field sales or call center transactions, it’s
almost impossible to know that someone
didn’t buy your product because of
price. Therefore, a realistic yet robust
WTP estimation for most service parts
manufacturers and distributors must
come from win-only data.
ESTIMATING WILLINGNESS-TO-PAY USING WIN-ONLY DATA
Let’s revisit the definition
of WTP: the highest price a
buyer (e.g., individual,
corporation, dealers,
distribution channel, etc.) is
willing to accept to pay for
some good or service.
Consider the price distribution for a
service part that sells between $1.00
and $3.00 as shown in Figure 1. If one
of your customers buys that product
for $1.45, then their WTP was greater
than or equal to that price. Since we
have only win data, that means the data
distribution is skewed as it relates to the
WTP of that customer or segment. Take
a closer look at Figure 1 — a specific
segment’s win distribution. Prices
cluster around $1.45. Does that mean
$1.45 is your best estimate of the WTP
of this segment?
Answer: No. It means that your sales
force, pricing desk and category
managers feel comfortable charging
around $1.45 per unit. The true WTP
of this segment is greater than $1.45.
So, what is the true WTP distribution
of this segment? Prescriptive pricing
technology can give you the answer.
PROS pricing science analyzes myriad
factors to statistically produce the best
possible estimate of WTP given the
winonly data typically available in
service parts markets. Based on the
example shown here and taking
into account part velocity, lifecycle
competitiveness and other key
transaction attributes, the Willingness-
to-Pay for this service part is best
estimated at $1.67. As one of the
key inputs into pricing optimization,
OPTIMIZING PRICING FOR THE AUTO/EQUIPMENT PARTS INDUSTRYOPTIMIZING PRICING FOR THE AUTO/EQUIPMENT PARTS INDUSTRY
98
accurate prediction of WTP plays a
large role in the quality of optimized
prices. An increase in pricing accuracy
can make a big impact on your
balance sheet. In implementation
after implementation, PROS customers
consistently observe a 100–300 basis
point gross profit improvement
compared to their corresponding
control groups by using sophisticated
pricing science.
DETERMINING WILLINGNESS-TO-PAY
HELPS BOOST PROFITS
Service parts manufacturers
and distributors consistently
customize and adjust their
pricing for good reasons.
Certain products demand a premium
over others. Different regions have
varying competitive landscapes.
Contracts expire under varying
circumstances. Whether you rely on a
centralized price matrix with
customized exceptions or a totally
autonomous sales force, understanding
the Willingness-to-Pay of your
customers under various conditions
can be a source of significantly
increased profits. And, the use of
new software technology based on
scientific analytics can help you exploit
this opportunity in a practical and
affordable way.
At PROS, we offer proven pricing
technology and an unmatched track
record of success in helping service
parts manufacturers and distributors
achieve outstanding returns through
a greater understanding of their
customers’ WTP.
You can learn more by visiting our
website at www.pros.com or by
emailing us at [email protected].
OPTIMIZING PRICING FOR THE AUTO/EQUIPMENT PARTS INDUSTRYOPTIMIZING PRICING FOR THE AUTO/EQUIPMENT PARTS INDUSTRY
98
REFERENCES
1. Breidert, C. (2005). Estimation
of willingness-to-pay. Theory,
measurement, and application.
Vienna: Doctoral Thesis, WU Vienna
University of Economics and
Business.
2. Breidert, C., Hahsler, M., &
Reutterer, T. (2006). A Review of
Methods for Measuring Willingness-
to-Pay. Innovative Marketing, 8-32.
3. Ferguson, M., & Agrawal, V. (2007).
Bid-response models for
customised pricing. Journal of
Revenue and Pricing Management,
212–228.
4. Leonhardt, D. (2010, November
10). The Enduring Myth of Gold’s
Record High. The New York
Times, p. B1.
5. Nagle, T., & Holden, R. (2002).
The Strategy and Tactics of Pricing.
Prentice Hall.
6. Phillips, R. (2005). Pricing and
Revenue Optimization. Stanford
Businesson scientific analytics can
help you exploit this opportunity in
a practical and affordable way.
OPTIMIZING PRICING FOR THE AUTO/EQUIPMENT PARTS INDUSTRYOPTIMIZING PRICING FOR THE AUTO/EQUIPMENT PARTS INDUSTRY
1110
ABOUT THE AUTHOR
Sean Duclaux serves as PROS Director,
Industry Marketing. He is responsible
for the development of the company’s
go-to-market strategy, and positioning
of its Service Parts pricing and revenue
management product portfolio.
Prior to joining PROS, Duclaux held
leadership positions in enterprise software
companies, including AspenTech, BMC
Software and Empirix. Throughout his
career, he has worked in diverse roles, from
product management, marketing, program
management, R&D and operations, where
he developed a strategic vision to define
market-focused solutions and executed go-
to-market programs.
Duclaux earned an MBA from the
University of Houston; an M.S. in computer
science from the University of New Orleans
and a B.S. from Spring Hill College.
OPTIMIZING PRICING FOR THE AUTO/EQUIPMENT PARTS INDUSTRYOPTIMIZING PRICING FOR THE AUTO/EQUIPMENT PARTS INDUSTRY
1110
Copyright © 2014, PROS Inc. All rights reserved. This document is provided for information purposes only and the contents hereof are subject to change without notice. This
document is not warranted to be error -free, nor subject to any other warranties or conditions, whether expressed orally or implied in law, including implied warranties and
conditions of merchantability or fitness for a particular purpose. We specifically disclaim any liability with respect to this document and no contractual obligations are formed
either directly or indirectly by this document. This document may not be reproduced or transmitted in any form or by any means, electronic or mechanical, for any purpose,
without our prior written permission.
About PROS
PROS.com
Copyright © 2015, PROS Inc. All rights reserved. This document is provided for information purposes only and the contents hereof are subject to change without notice. This
document is not warranted to be error -free, nor subject to any other warranties r conditions, whether expresse orally or implied in l w, including implied arranties and
conditions of merchantability or fitness for a particular purpose. We specifically disclaim any liability with respect to this document and o contractual obligations are formed
either irectly or indirectly y this document. This document may not be reproduced or transmitted in any form or by any means, lectronic or mechanical, for any purpose,
without our prior written permission.
PROS Holdings, Inc. (NYSE: PRO) is a big data software company that
helps customers outperform in their markets by using big data to sell
more effectively. We apply years of data science experience to unlock
buying patterns and preferences within transaction data to reveal which
opportunities are most likely to close, which offers are most likely to sell
and which prices are most likely to win. PROS offers big data solutions to
optimize sales, pricing, quoting, rebates and revenue management
across more than 40 industries. PROS has completed over 800
implementations of its solutions in more than 55 countries. The PROS
team comprises approximately 1,000 professionals around the world.
To learn more, visit pros.com.