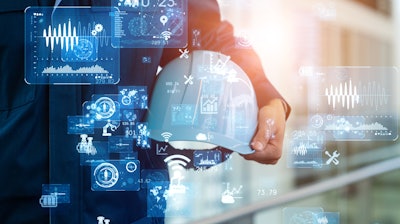
In a recent report by Appinventiv, 66 percent of manufacturers incorporating AI into their daily operations report a growing dependence on the technology, signaling the need to take proactive steps to ensure organizational security.
Through the integration of AI, these organizations are experiencing increased innovation, cost savings and productivity. However, without comprehensive security and compliance measures, they are leaving themselves vulnerable to risks such as inaccurate outputs, security vulnerabilities and regulatory missteps that can easily culminate in financial and legal consequences. Manufacturers who assess their AI governance and adopt a proactive approach are better positioned to ensure that the technology is working for them, rather than against them.
In this surge of AI application, manufacturers are using AI-powered tools to employ predictive maintenance, monitor supply chains in real-time and enhance quality control. According to The National Association of Manufacturers 2024 report 72 percent of manufacturers using these techniques have reported reduced costs and improved operational efficiency. Despite this, the rapid adoption of AI without guardrails can quickly take a turn to be more harmful than helpful.
With the race to modernize operations and keep up with competitors, it’s easy for businesses to skip past establishing the proper governance for their new technology. Though executives are beginning to make plans to create AI governance frameworks, 95 percent have yet to implement said frameworks to mitigate risk.
Speeding through this important step in adopting AI creates major security vulnerabilities that can easily undo any forward movement the technology has made, possibly inviting massive setbacks, including regulatory penalties, cyberattacks and the disruption of operations.
Navigating Compliance, Security and Accuracy Risks
Amid the industry’s current labor crisis, the surge in automation has raised concerns for manufacturers around job availability. Research from McKinsey estimates that up to 800 million people could be affected by AI automation by 2030. Without human oversight, other potential risks generated from AI’s deployment include:
- Weakened Security Posture. AI systems used in manufacturing are often responsible for handling and processing large amounts of proprietary data, such as product designs, techniques and supply chain logistics. These AI models are vulnerable to cyberattacks, seen in threat actors injecting false data and compromising manufacturer’s decision-making processes. AI also has the ability to empower bad actors through deepfake technology and AI-generated phishing attacks. Hackers can exploit AI-driven assistants and trick employees into revealing sensitive information, making AI both a tool and a weapon.
- Impaired Decision-Making. AI models are easily misguided if the data given to them is incomplete, biased or outdated, which leads to flawed outputs. When organizations provide internal data to support their AI models in producing accurate outputs, such as product defect detection or supply chain forecasts, inaccurate data could easily lead to more waste, increased recalls and even regulatory action. Industry leaders should be cognizant of their reliance on AI, ensure human oversight, and conduct consistent validation and auditing of their AI tools to maintain the necessary accuracy and integrity.
- Regulatory Misalignment. AI-specific compliance regulations are emerging as more industries adopt and integrate AI into their industrial operations. Some of these requirements include mandates for transparency, data privacy and accountability in AI decision-making. Noncompliance with these standards results in severe legal penalties for manufacturers in addition to operational restrictions. While the U.S. is still developing AI-specific regulation, in relation to other nations further along in the process, there are privacy and data protections currently in place in the U.S. that cover AI operations and tools. To ensure compliance, manufacturers should align their governance strategies accordingly and invest in compliance tracking and reporting to stay up to date as further protections pass to legislation.
Organizations should consider adopting a comprehensive, proactive governance approach to mitigate AI risks. This is best done through instituting policies for the development and management of AI-powered tools, monitoring its deployment and integrating security and compliance measures. Other strategies that can help safeguard AI investments in manufacturing include:
Centralized Risk Management
A centralized governance, risk and compliance (GRC) system provides manufacturers with a comprehensive outlook of potential risks across all departments. This framework enables consistent tracking and enforcement of standardized controls, providing AI-related risk information pertaining to production, quality control and supply chain management in covering the following:
- Risk assessment frameworks must be able to identify potential vulnerabilities and subsequent consequences, such as AI model bias and low-quality data.
- AI-driven security breaches often differ from traditional IT breaches, meaning incident response plans must cover AI-specific procedures such as containment, eradication, recovery and post-incident analysis.
- Detailed documentation of data sources, training processes, and validation results ensures internal accountability and is often required to maintain compliance (such as GDPR and CCPA compliance).
Automated Compliance Monitoring
Ongoing and evolving regulatory compliance standards require real-time compliance and reporting. Automated compliance tools allow organizations to:
- Evaluate compliance status with full visibility and key metrics.
- Provide generated and formatted regulatory adherence reports to stakeholders.
- Immediately notify executives and stakeholders of potential compliance risks before they intensify.
Ongoing Data Validation and Model Auditing
Since GenAI systems require large amounts of data to learn from, AI outputs require intense scrutiny to ensure data privacy and integrity, as well as adherence to fairness, bias and regulatory requirements. When auditing AI models, business best practices include:
- Checking for AI biases and inaccuracies by testing systems against real-world scenarios.
- Maintaining updated data training sets, reflecting current industry conditions.
- Developing processes for human experts to review AI decisions for accuracy
Cybersecurity-First AI Deployment
AI systems process large amounts of sensitive data that makes a valuable target for cybercriminals. Organizations should seek a proactive, cybersecurity-first approach when deploying AI as it becomes more embedded in operational activities. Key tactics for this include:
- Systematically monitoring data and processes associated with organizational AI systems.
- Utilizing multi-factor authentication and encryption processes in AI tools and data to prevent unauthorized access to sensitive information.
- Allowing only verified datasets in AI model training to reduce manipulation risks.
- Integrating custom guardrails to prevent AI bias, ensure data privacy and regulatory compliance and eliminate harmful AI outputs.
Without a proactive approach, manufacturers expose their operations to major security threats and expensive compliance violations that effectively discredit any innovation or efficiency that AI-powered tools hope to bring. By establishing AI governance frameworks in a centralized GRC system, manufacturers can achieve a reliable, compliant and secure modernization of their supply chains, which aids in maintaining competitiveness in a rapidly evolving industry.