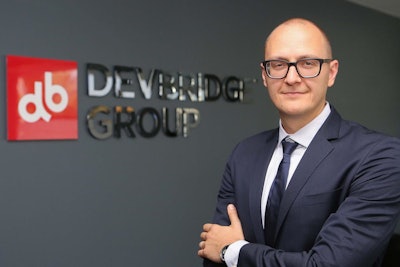
Leveraging Big Data to predict downtime on the manufacturing floor is something we’ve been spending a lot of time on in our labs. In reality, interpreting Big Data in manufacturing is a highly complex process that involves an interconnected fleet of data producing machines, overwhelming amounts of data, transformation of data for digestion, interpretation and automation of strategic decisions to maximize uptime and profitability.
Collecting Big Data
The Internet of Things — a term used to describe the interconnectivity of all smart devices — in this case applies to the machines on a manufacturing floor, the computers, smartphones and tablets on desks or pockets, and everything in between. A connection and a standardized interface between all of these devices allow for the transmission and collection of large amounts of data. The standard that makes this possible in manufacturing is called MTConnect.
MTConnect is an open channel of communication that provides plug-and-play interconnectivity to facilitate real-time data exchange between machines and devices using XML and HTTP. Using MTConnect’s OpenSource format, manufacturers can continuously retrieve valuable operational data from their own fleet of machines. The sort of data that is received and ultimately analyzed through MTConnect includes temperatures, revolutions per minutes on spindles and machine activity, among other things. Collecting and transforming this information is step one, but you can see where this data – and the ability to interpret this data– is going to be highly valuable from an operational viewpoint.
Interpreting Big Data
As it stands, the sheer amount of events and the way the data is collected makes it difficult for us to do anything with it. The industry has responded with software that helps analyze and simplify the data, allowing us to digest and contextualize what we’re seeing. After all, without context and knowing what to look for the data is largely meaningless. We’re talking about incredibly large amounts of data; a large shop with 100 machines or tools can produce terabytes of monitoring data annually.
Using visualization tools similar to, for example, Kibana, which is a dynamic interface for making sense of data on an ElasticSearch database, predictive analytics can be built. With this information, you can pinpoint and forecast machine malfunctions quickly so as to minimize the potential production downtime. Additionally, the data collected through MTConnect allows machines to be proactively serviced, leading to increased uptime, lowered manufacturing costs, and higher profitability.
Automation can be pushed even further. RFID chips can be embedded on machine parts that allow maintenance personnel to easily scan and order replacements without having deep technical knowledge of machine operation. By scanning the chip, an alert is sent out to place an automated order via an iOS application. Collecting failure and replacement data allows us to build predictive analytics and schedule automatic re-ordering of parts that have rated wear and tear duration. All of this is just a scratch on the surface of data driven operational optimization.
A precursor for this technology, for example, has been at work in the IT industry for some time. IBM has been proactively replacing the hardware components of their servers before they fail using technologies they originally termed “Predictive Failure Analysis” and “environmental monitoring” since the early 1990s. Predictive Failure Analysis would go on to become a widespread technology used for hard-disk and solid-state drive monitoring called Self-Monitoring, Analysis and Reporting Technology, or SMART.
Conclusion
Creating predictive analytics based on data collected by field-deployed smart devices is already a massive trend across multiple industries. Leading software and service providers have recognized the importance of Big Data and are gearing up for the demand with new products and services. Microsoft has recently added Internet of Things and Big Data orchestration services to their Microsoft Azure Cloud offerings. SAP, IBM and other companies are also offering services and software related to Big Data. Manufacturers are just now beginning to realize the power they can potentially yield through the data they collect form their machines and tools and we’re thrilled to be at the forefront, developing algorithms and applications that will allow them to further optimize their process.
Aurimas Adomavicius and his partners founded Devbridge Group in 2008 based on the beliefs that software should go hand in hand with intuitive and elegant user experience.